Open Access
ARTICLE
Improving Routine Immunization Coverage Through Optimally Designed Predictive Models
1 Faculty of Electrical and Computer Engineering, NED University of Engineering and Technology, Karachi, 75270, Pakistan
2 Department of Pediatrics and Child Health, Aga Khan University, Karachi, 74800, Pakistan
3 Surrey Business School, University of Surrey, Guildford, GU2 7XH, United Kingdom
4 Neurocomputation Lab, National Centre of Artificial Intelligence, NED University of Engineering &Technology, Karachi, 75270, Pakistan
5 Faculty of IT and Design, The Hague University of Applied Sciences, 2521 EN, The Hague, The Netherlands
* Corresponding Author: Majida Kazmi. Email:
Computers, Materials & Continua 2022, 70(1), 375-395. https://doi.org/10.32604/cmc.2022.019167
Received 04 April 2021; Accepted 08 May 2021; Issue published 07 September 2021
Abstract
Routine immunization (RI) of children is the most effective and timely public health intervention for decreasing child mortality rates around the globe. Pakistan being a low-and-middle-income-country (LMIC) has one of the highest child mortality rates in the world occurring mainly due to vaccine-preventable diseases (VPDs). For improving RI coverage, a critical need is to establish potential RI defaulters at an early stage, so that appropriate interventions can be targeted towards such population who are identified to be at risk of missing on their scheduled vaccine uptakes. In this paper, a machine learning (ML) based predictive model has been proposed to predict defaulting and non-defaulting children on upcoming immunization visits and examine the effect of its underlying contributing factors. The predictive model uses data obtained from Paigham-e-Sehat study having immunization records of 3,113 children. The design of predictive model is based on obtaining optimal results across accuracy, specificity, and sensitivity, to ensure model outcomes remain practically relevant to the problem addressed. Further optimization of predictive model is obtained through selection of significant features and removing data bias. Nine machine learning algorithms were applied for prediction of defaulting children for the next immunization visit. The results showed that the random forest model achieves the optimal accuracy of 81.9% with 83.6% sensitivity and 80.3% specificity. The main determinants of vaccination coverage were found to be vaccine coverage at birth, parental education, and socio-economic conditions of the defaulting group. This information can assist relevant policy makers to take proactive and effective measures for developing evidence based targeted and timely interventions for defaulting children.Keywords
Cite This Article
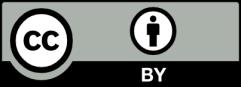
This work is licensed under a Creative Commons Attribution 4.0 International License , which permits unrestricted use, distribution, and reproduction in any medium, provided the original work is properly cited.