Open Access
ARTICLE
Deep Semisupervised Learning-Based Network Anomaly Detection in Heterogeneous Information Systems
1 Lviv Polytechnic National University, Lviv, 79013, Ukraine
2 West Ukrainian National University, Ternopil, 46009, Ukraine
3 Technical University of Kosice, Kosice, 04200, Slovakia
* Corresponding Author: Taras Maksymyuk. Email:
Computers, Materials & Continua 2022, 70(1), 413-431. https://doi.org/10.32604/cmc.2022.018773
Received 21 March 2021; Accepted 23 April 2021; Issue published 07 September 2021
Abstract
The extensive proliferation of modern information services and ubiquitous digitization of society have raised cybersecurity challenges to new levels. With the massive number of connected devices, opportunities for potential network attacks are nearly unlimited. An additional problem is that many low-cost devices are not equipped with effective security protection so that they are easily hacked and applied within a network of bots (botnet) to perform distributed denial of service (DDoS) attacks. In this paper, we propose a novel intrusion detection system (IDS) based on deep learning that aims to identify suspicious behavior in modern heterogeneous information systems. The proposed approach is based on a deep recurrent autoencoder that learns time series of normal network behavior and detects notable network anomalies. An additional feature of the proposed IDS is that it is trained with an optimized dataset, where the number of features is reduced by 94% without classification accuracy loss. Thus, the proposed IDS remains stable in response to slight system perturbations, which do not represent network anomalies. The proposed approach is evaluated under different simulation scenarios and provides a 99% detection accuracy over known datasets while reducing the training time by an order of magnitude.Keywords
Cite This Article
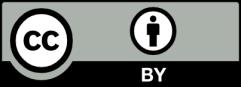
This work is licensed under a Creative Commons Attribution 4.0 International License , which permits unrestricted use, distribution, and reproduction in any medium, provided the original work is properly cited.