Open Access
ARTICLE
Automated COVID-19 Detection Based on Single-Image Super-Resolution and CNN Models
1 Department Electronics and Electrical Communications, Faculty of Electronic Engineering, Menoufia University, Menouf, 32952, Egypt
2 Alexandria Higher Institute of Engineering & Technology (AIET), Alexandria, Egypt
3 Department of Information Technology, College of Computer and Information Sciences, Princess Nourah Bint Abdulrahman University, Riyadh, 84428, Saudi Arabia
* Corresponding Author: Naglaa F. Soliman. Email:
Computers, Materials & Continua 2022, 70(1), 1141-1157. https://doi.org/10.32604/cmc.2022.018547
Received 12 March 2021; Accepted 26 May 2021; Issue published 07 September 2021
Abstract
In developing countries, medical diagnosis is expensive and time consuming. Hence, automatic diagnosis can be a good cheap alternative. This task can be performed with artificial intelligence tools such as deep Convolutional Neural Networks (CNNs). These tools can be used on medical images to speed up the diagnosis process and save the efforts of specialists. The deep CNNs allow direct learning from the medical images. However, the accessibility of classified data is still the largest challenge, particularly in the field of medical imaging. Transfer learning can deliver an effective and promising solution by transferring knowledge from universal object detection CNNs to medical image classification. However, because of the inhomogeneity and enormous overlap in intensity between medical images in terms of features in the diagnosis of Pneumonia and COVID-19, transfer learning is not usually a robust solution. Single-Image Super-Resolution (SISR) can facilitate learning to enhance computer vision functions, apart from enhancing perceptual image consistency. Consequently, it helps in showing the main features of images. Motivated by the challenging dilemma of Pneumonia and COVID-19 diagnosis, this paper introduces a hybrid CNN model, namely SIGTra, to generate super-resolution versions of X-ray and CT images. It depends on a Generative Adversarial Network (GAN) for the super-resolution reconstruction problem. Besides, Transfer learning with CNN (TCNN) is adopted for the classification of images. Three different categories of chest X-ray and CT images can be classified with the proposed model. A comparison study is presented between the proposed SIGTra model and the other related CNN models for COVID-19 detection in terms of precision, sensitivity, and accuracy.Keywords
Cite This Article
Citations
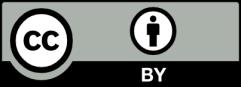
This work is licensed under a Creative Commons Attribution 4.0 International License , which permits unrestricted use, distribution, and reproduction in any medium, provided the original work is properly cited.