Open Access
ARTICLE
Optimized Convolutional Neural Network for Automatic Detection of COVID-19
1 Department of Information Technology, PSNA College of Engineering and Technology, Dindigul, 624622, Tamilnadu, India
2 Department of Computer Science & Engineering, PSNA College of Engineering and Technology, Dindigul, 624622, India
3 School of Computing, SASTRA Deemed University, Thanjavur, 613401, India
4 Univ. Artois, U. R. 3926, Laboratoire de Génie Informatique et d’Automatique de l’Artois (LGI2A), F-62400, Béthune, France
5 Department of Mathematics, Faculty of Science, New Valley University, El-Kharga, 72511, Egypt
* Corresponding Author: Adnen El Amraoui. Email:
Computers, Materials & Continua 2022, 70(1), 1159-1175. https://doi.org/10.32604/cmc.2022.017178
Received 23 January 2021; Accepted 24 April 2021; Issue published 07 September 2021
Abstract
The outbreak of COVID-19 affected global nations and is posing serious challenges to healthcare systems across the globe. Radiologists use X-Rays or Computed Tomography (CT) images to confirm the presence of COVID-19. So, image processing techniques play an important role in diagnostic procedures and it helps the healthcare professionals during critical times. The current research work introduces Multi-objective Black Widow Optimization (MBWO)-based Convolutional Neural Network i.e., MBWO-CNN technique for diagnosis and classification of COVID-19. MBWO-CNN model involves four steps such as preprocessing, feature extraction, parameter tuning, and classification. In the beginning, the input images undergo preprocessing followed by CNN-based feature extraction. Then, Multi-objective Black Widow Optimization (MBWO) technique is applied to fine tune the hyperparameters of CNN. Finally, Extreme Learning Machine with autoencoder (ELM-AE) is applied as a classifier to confirm the presence of COVID-19 and classify the disease under different class labels. The proposed MBWO-CNN model was validated experimentally and the results obtained were compared with the results achieved by existing techniques. The experimental results ensured the superior results of the ELM-AE model by attaining maximum classification performance with the accuracy of 96.43%. The effectiveness of the technique is proved through promising results and the model can be applied in diagnosis and classification of COVID-19.Keywords
Cite This Article
Citations
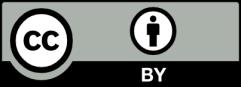
This work is licensed under a Creative Commons Attribution 4.0 International License , which permits unrestricted use, distribution, and reproduction in any medium, provided the original work is properly cited.