Open Access
ARTICLE
A Multi-Feature Learning Model with Enhanced Local Attention for Vehicle Re-Identification
1 Jiangsu Collaborative Innovation Center of Atmospheric Environment and Equipment Technology, Nanjing University of Information Science & Technology, Nanjing, 210044, China
2 School of Automation, Nanjing University of Information Science & Technology, Nanjing, 210044, China
3 Engineering Research Center of Digital Forensics, Ministry of Education, Jiangsu Engineering Center of Network Monitoring, School of Computer and Software, Nanjing University of Information Science & Technology, Nanjing, 210044, China
4 Rensselaer Polytechnic Institute, Troy, NY, 12180, USA
* Corresponding Author: Wei Sun. Email:
Computers, Materials & Continua 2021, 69(3), 3549-3561. https://doi.org/10.32604/cmc.2021.021627
Received 29 June 2021; Accepted 30 July 2021; Issue published 24 August 2021
Abstract
Vehicle re-identification (ReID) aims to retrieve the target vehicle in an extensive image gallery through its appearances from various views in the cross-camera scenario. It has gradually become a core technology of intelligent transportation system. Most existing vehicle re-identification models adopt the joint learning of global and local features. However, they directly use the extracted global features, resulting in insufficient feature expression. Moreover, local features are primarily obtained through advanced annotation and complex attention mechanisms, which require additional costs. To solve this issue, a multi-feature learning model with enhanced local attention for vehicle re-identification (MFELA) is proposed in this paper. The model consists of global and local branches. The global branch utilizes both middle and high-level semantic features of ResNet50 to enhance the global representation capability. In addition, multi-scale pooling operations are used to obtain multi-scale information. While the local branch utilizes the proposed Region Batch Dropblock (RBD), which encourages the model to learn discriminative features for different local regions and simultaneously drops corresponding same areas randomly in a batch during training to enhance the attention to local regions. Then features from both branches are combined to provide a more comprehensive and distinctive feature representation. Extensive experiments on VeRi-776 and VehicleID datasets prove that our method has excellent performance.Keywords
Cite This Article
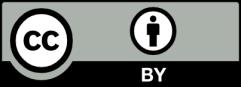