Open Access
ARTICLE
Hybrid Neural Network for Automatic Recovery of Elliptical Chinese Quantity Noun Phrases
1 School of Computer Science and Technology, Nanjing Normal University, Nanjing, 210023, China
2 School of Chinese Language and Literature, Nanjing Normal University, Nanjing, 210097, China
3 International College for Chinese Studies, Nanjing Normal University, Nanjing, 210097, China
4 School of Computer Science and Electronic Engineering, University of Essex, Colchester, UK
* Corresponding Author: Weiguang Qu. Email:
Computers, Materials & Continua 2021, 69(3), 4113-4127. https://doi.org/10.32604/cmc.2021.019518
Received 15 April 2021; Accepted 04 June 2021; Issue published 24 August 2021
Abstract
In Mandarin Chinese, when the noun head appears in the context, a quantity noun phrase can be reduced to a quantity phrase with the noun head omitted. This phrase structure is called elliptical quantity noun phrase. The automatic recovery of elliptical quantity noun phrase is crucial in syntactic parsing, semantic representation and other downstream tasks. In this paper, we propose a hybrid neural network model to identify the semantic category for elliptical quantity noun phrases and realize the recovery of omitted semantics by supplementing concept categories. Firstly, we use BERT to generate character-level vectors. Secondly, Bi-LSTM is applied to capture the context information of each character and compress the input into the context memory history. Then CNN is utilized to capture the local semantics of n-grams with various granularities. Based on the Chinese Abstract Meaning Representation (CAMR) corpus and Xinhua News Agency corpus, we construct a hand-labeled elliptical quantity noun phrase dataset and carry out the semantic recovery of elliptical quantity noun phrase on this dataset. The experimental results show that our hybrid neural network model can effectively improve the performance of the semantic complement for the elliptical quantity noun phrases.Keywords
Cite This Article
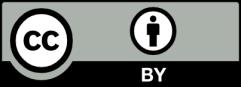