Open Access
ARTICLE
Entropy Bayesian Analysis for the Generalized Inverse Exponential Distribution Based on URRSS
1 Faculty of Science, Department of Mathematics, Al al-Bayt University, Mafraq, 25113, Jordan
2 Faculty of Graduate Studies for Statistical Research, Cairo University, Giza, 12613, Egypt
3 Department of Mathematics, College of Science and Human Studies at Hotat Sudair, Majmaah University, Majmaah, 11952, Saudia Arabia
* Corresponding Author: Ayed R. A. Al-Anzi. Email:
Computers, Materials & Continua 2021, 69(3), 3795-3811. https://doi.org/10.32604/cmc.2021.019061
Received 31 March 2021; Accepted 03 May 2021; Issue published 24 August 2021
Abstract
This paper deals with the Bayesian estimation of Shannon entropy for the generalized inverse exponential distribution. Assuming that the observed samples are taken from the upper record ranked set sampling (URRSS) and upper record values (URV) schemes. Formulas of Bayesian estimators are derived depending on a gamma prior distribution considering the squared error, linear exponential and precautionary loss functions, in addition, we obtain Bayesian credible intervals. The random-walk Metropolis-Hastings algorithm is handled to generate Markov chain Monte Carlo samples from the posterior distribution. Then, the behavior of the estimates is examined at various record values. The output of the study shows that the entropy Bayesian estimates under URRSS are more convenient than the other estimates under URV in the majority of the situations. Also, the entropy Bayesian estimates perform well as the number of records increases. The obtained results validate the usefulness and efficiency of the URV method. Real data is analyzed for more clarifying purposes which validate the theoretical results.Keywords
Cite This Article
Citations
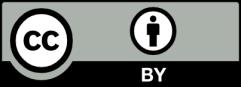
This work is licensed under a Creative Commons Attribution 4.0 International License , which permits unrestricted use, distribution, and reproduction in any medium, provided the original work is properly cited.