Open Access
ARTICLE
Convolutional Neural Network for Histopathological Osteosarcoma Image Classification
1 Center of Excellence in Information Technology, Institute of Management Sciences, Peshawar, Pakistan
2 Department of Computer Sciences, College of Computer and Information Sciences, Princess Nourah Bint Abdulrahman University (PNU), Riyadh, Saudi Arabia
* Corresponding Author: Imran Ahmed. Email:
(This article belongs to the Special Issue: Security and Privacy issues for various Emerging Technologies and Future Trends)
Computers, Materials & Continua 2021, 69(3), 3365-3381. https://doi.org/10.32604/cmc.2021.018486
Received 10 March 2021; Accepted 30 April 2021; Issue published 24 August 2021
Abstract
Osteosarcoma is one of the most widespread causes of bone cancer globally and has a high mortality rate. Early diagnosis may increase the chances of treatment and survival however the process is time-consuming (reliability and complexity involved to extract the hand-crafted features) and largely depends on pathologists’ experience. Convolutional Neural Network (CNN—an end-to-end model) is known to be an alternative to overcome the aforesaid problems. Therefore, this work proposes a compact CNN architecture that has been rigorously explored on a Small Osteosarcoma histology Image Dataaseet (a high-class imbalanced dataset). Though, during training, class-imbalanced data can negatively affect the performance of CNN. Therefore, an oversampling technique has been proposed to overcome the aforesaid issue and improve generalization performance. In this process, a hierarchical CNN model is designed, in which the former model is non-regularized (due to dense architecture) and the later one is regularized, specifically designed for small histopathology images. Moreover, the regularized model is integrated with CNN’s basic architecture to reduce overfitting. Experimental results demonstrate that oversampling might be an effective way to address the imbalanced class problem during training. The training and testing accuracies of the non-regularized CNN model are 98% & 78% with an imbalanced dataset and 96% & 81% with a balanced dataset, respectively. The regularized CNN model training and testing accuracies are 84% & 75% for an imbalanced dataset and 87% & 86% for a balanced dataset.Keywords
Cite This Article
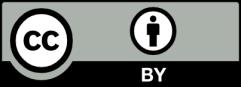
This work is licensed under a Creative Commons Attribution 4.0 International License , which permits unrestricted use, distribution, and reproduction in any medium, provided the original work is properly cited.