Open Access
ARTICLE
An AW-HARIS Based Automated Segmentation of Human Liver Using CT Images
1 Department of Computer Science and Engineering, GITAM Institute of Technology, GITAM Deemed to be University, Visakhapatnam, 530045, India
2 Department of Computer Science, College of Computer Sciences and Information Technology, King Faisal University, Al-Ahsa, 31982, Saudi Arabia
3 Department of Electrical and Electronics Engineering, Sikkim Manipal Institute of Technology, Sikkim Manipal University, Majitar, 737136, India
4 Department of Intelligent Mechatronics Engineering, Sejong University, Seoul, 05006, Korea
* Corresponding Author: Shakeel Ahmed. Email:
Computers, Materials & Continua 2021, 69(3), 3303-3319. https://doi.org/10.32604/cmc.2021.018472
Received 10 March 2021; Accepted 25 April 2021; Issue published 24 August 2021
Abstract
In the digestion of amino acids, carbohydrates, and lipids, as well as protein synthesis from the consumed food, the liver has many diverse responsibilities and functions that are to be performed. Liver disease may impact the hormonal and nutritional balance in the human body. The earlier diagnosis of such critical conditions may help to treat the patient effectively. A computationally efficient AW-HARIS algorithm is used in this paper to perform automated segmentation of CT scan images to identify abnormalities in the human liver. The proposed approach can recognize the abnormalities with better accuracy without training, unlike in supervisory procedures requiring considerable computational efforts for training. In the earlier stages, the CT images are pre-processed through an Adaptive Multiscale Data Condensation Kernel to normalize the underlying noise and enhance the image’s contrast for better segmentation. Then, the preliminary phase’s outcome is being fed as the input for the Anisotropic Weighted–-Heuristic Algorithm for Real-time Image Segmentation algorithm that uses texture-related information, which has resulted in precise outcome with acceptable computational latency when compared to that of its counterparts. It is observed that the proposed approach has outperformed in the majority of the cases with an accuracy of 78%. The smart diagnosis approach would help the medical staff accurately predict the abnormality and disease progression in earlier ailment stages.Keywords
Cite This Article
Citations
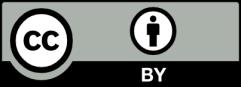
This work is licensed under a Creative Commons Attribution 4.0 International License , which permits unrestricted use, distribution, and reproduction in any medium, provided the original work is properly cited.