Open Access
ARTICLE
Automatic Detection of COVID-19 Using a Stacked Denoising Convolutional Autoencoder
1 College of Applied Computer Sciences (CACS), King Saud University, Riyadh, 11543, Saudi Arabia
2 Faculty of Sciences and Technology of Sidi Bouzid, University of Kairouan, Kairouan, Tunisia
3 National School of Engineers (ENIS), University of Sfax, Tunisia
4 Department of Computer Sciences, College of Sciences and Arts in Unaizah, Qassim University, Al-Qassim, Kingdom of Saudi Arabia
* Corresponding Author: Habib Dhahri. Email:
(This article belongs to the Special Issue: Emerging Trends in Artificial Intelligence and Machine Learning)
Computers, Materials & Continua 2021, 69(3), 3259-3274. https://doi.org/10.32604/cmc.2021.018449
Received 09 March 2021; Accepted 15 April 2021; Issue published 24 August 2021
Abstract
The exponential increase in new coronavirus disease 2019 ({COVID-19}) cases and deaths has made COVID-19 the leading cause of death in many countries. Thus, in this study, we propose an efficient technique for the automatic detection of COVID-19 and pneumonia based on X-ray images. A stacked denoising convolutional autoencoder (SDCA) model was proposed to classify X-ray images into three classes: normal, pneumonia, and {COVID-19}. The SDCA model was used to obtain a good representation of the input data and extract the relevant features from noisy images. The proposed model’s architecture mainly composed of eight autoencoders, which were fed to two dense layers and SoftMax classifiers. The proposed model was evaluated with 6356 images from the datasets from different sources. The experiments and evaluation of the proposed model were applied to an 80/20 training/validation split and for five cross-validation data splitting, respectively. The metrics used for the SDCA model were the classification accuracy, precision, sensitivity, and specificity for both schemes. Our results demonstrated the superiority of the proposed model in classifying X-ray images with high accuracy of 96.8%. Therefore, this model can help physicians accelerate COVID-19 diagnosis.Keywords
Cite This Article
Citations
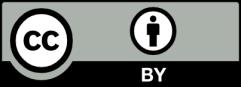
This work is licensed under a Creative Commons Attribution 4.0 International License , which permits unrestricted use, distribution, and reproduction in any medium, provided the original work is properly cited.