Open Access
ARTICLE
Pseudo Zernike Moment and Deep Stacked Sparse Autoencoder for COVID-19 Diagnosis
1 School of Informatics, University of Leicester, Leicester, LE1 7RH, UK
2 Department of Computer Science, HITEC University Taxila, Taxila, Pakistan
3 Science in Civil Engineering, University of Florida, Gainesville, Florida, FL 32608, Gainesville, USA
4 School of Mathematics and Actuarial Science, University of Leicester, LE1 7RH, UK
* Corresponding Author: Shui-Hua Wang. Email:
(This article belongs to the Special Issue: Recent Advances in Deep Learning for Medical Image Analysis)
Computers, Materials & Continua 2021, 69(3), 3145-3162. https://doi.org/10.32604/cmc.2021.018040
Received 22 February 2021; Accepted 07 April 2021; Issue published 24 August 2021
Abstract
(Aim) COVID-19 is an ongoing infectious disease. It has caused more than 107.45 m confirmed cases and 2.35 m deaths till 11/Feb/2021. Traditional computer vision methods have achieved promising results on the automatic smart diagnosis. (Method) This study aims to propose a novel deep learning method that can obtain better performance. We use the pseudo-Zernike moment (PZM), derived from Zernike moment, as the extracted features. Two settings are introducing: (i) image plane over unit circle; and (ii) image plane inside the unit circle. Afterward, we use a deep-stacked sparse autoencoder (DSSAE) as the classifier. Besides, multiple-way data augmentation is chosen to overcome overfitting. The multiple-way data augmentation is based on Gaussian noise, salt-and-pepper noise, speckle noise, horizontal and vertical shear, rotation, Gamma correction, random translation and scaling. (Results) 10 runs of 10-fold cross validation shows that our PZM-DSSAE method achieves a sensitivity of 92.06% ± 1.54%, a specificity of 92.56% ± 1.06%, a precision of 92.53% ± 1.03%, and an accuracy of 92.31% ± 1.08%. Its F1 score, MCC, and FMI arrive at 92.29% ±1.10%, 84.64% ± 2.15%, and 92.29% ± 1.10%, respectively. The AUC of our model is 0.9576. (Conclusion) We demonstrate “image plane over unit circle” can get better results than “image plane inside a unit circle.” Besides, this proposed PZM-DSSAE model is better than eight state-of-the-art approaches.Keywords
Cite This Article
Citations
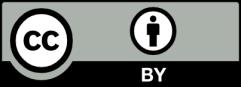