Open Access
ARTICLE
Classification of Retroviruses Based on Genomic Data Using RVGC
1 Department of CS & IT, University of Sargodha, Sargodha, 40100, Pakistan
2 Department of CS & IT, University of Mianwali, Mianwali, 42200, Pakistan
3 School of Systems and Technology, University of Management and Technology, Lahore, 54782, Pakistan
4 Department of Computer Science, HITEC University Taxila, Taxila, Pakistan
5 Department of Computer Science and Engineering, Soonchunhyang University, Asan, Korea
6 Faculty of Applied Computing and Technology, Noroff University College, Kristiansand, Norway
* Corresponding Author: Yunyoung Nam. Email:
(This article belongs to the Special Issue: Recent Advances in Deep Learning for Medical Image Analysis)
Computers, Materials & Continua 2021, 69(3), 3829-3844. https://doi.org/10.32604/cmc.2021.017835
Received 13 February 2021; Accepted 17 April 2021; Issue published 24 August 2021
Abstract
Retroviruses are a large group of infectious agents with similar virion structures and replication mechanisms. AIDS, cancer, neurologic disorders, and other clinical conditions can all be fatal due to retrovirus infections. Detection of retroviruses by genome sequence is a biological problem that benefits from computational methods. The National Center for Biotechnology Information (NCBI) promotes science and health by making biomedical and genomic data available to the public. This research aims to classify the different types of rotavirus genome sequences available at the NCBI. First, nucleotide pattern occurrences are counted in the given genome sequences at the preprocessing stage. Based on some significant results, the number of features used for classification is reduced to five. The classification shall be carried out in two phases. The first phase of classification shall select only two features. Unclassified data in the first phase is transferred to the next phase, where the final decision is taken with the remaining three features. Three data sets of animals and human retroviruses are selected; the training data set is used to minimize the classifier’s number and training; the validation data set is used to validate the models. The performance of the classifier is analyzed using the test data set. Also, we use decision tree, naive Bayes, k-nearest neighbors, and vector support machines to compare results. The results show that the proposed approach performs better than the existing methods for the retrovirus’s imbalanced genome-sequence dataset.Keywords
Cite This Article
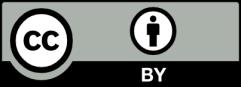
This work is licensed under a Creative Commons Attribution 4.0 International License , which permits unrestricted use, distribution, and reproduction in any medium, provided the original work is properly cited.