Open Access
ARTICLE
Cotton Leaf Diseases Recognition Using Deep Learning and Genetic Algorithm
1 Department of Computer Science, HITEC University Taxila, Taxila, Pakistan
2 Wah Engineering College, University of Wah, Wah Cantt, 47080, Pakistan
3 College of Computer Engineering and Sciences, Prince Sattam Bin Abdulaziz University, Al-Khraj, Saudi Arabia
4 Department of Computer Science and Engineering, Soonchunhyang University, Asan, Korea
5 Faculty of Applied Computing and Technology, Noroff University College, Kristiansand, Norway
* Corresponding Author: Yunyoung Nam. Email:
(This article belongs to the Special Issue: Recent Advances in Deep Learning and Saliency Methods for Agriculture)
Computers, Materials & Continua 2021, 69(3), 2917-2932. https://doi.org/10.32604/cmc.2021.017364
Received 28 January 2021; Accepted 14 April 2021; Issue published 24 August 2021
Abstract
Globally, Pakistan ranks 4 in cotton production, 6 as an importer of raw cotton, and 3 in cotton consumption. Nearly 10% of GDP and 55% of the country's foreign exchange earnings depend on cotton products. Approximately 1.5 million people in Pakistan are engaged in the cotton value chain. However, several diseases such as Mildew, Leaf Spot, and Soreshine affect cotton production. Manual diagnosis is not a good solution due to several factors such as high cost and unavailability of an expert. Therefore, it is essential to develop an automated technique that can accurately detect and recognize these diseases at their early stages. In this study, a new technique is proposed using deep learning architecture with serially fused features and the best feature selection. The proposed architecture consists of the following steps: (a) a self-collected dataset of cotton diseases is prepared and labeled by an expert; (b) data augmentation is performed on the collected dataset to increase the number of images for better training at the earlier step; (c) a pre-trained deep learning model named ResNet101 is employed and trained through a transfer learning approach; (d) features are computed from the third and fourth last layers and serially combined into one matrix; (e) a genetic algorithm is applied to the combined matrix to select the best points for further recognition. For final recognition, a Cubic SVM approach was utilized and validated on a prepared dataset. On the newly prepared dataset, the highest achieved accuracy was 98.8% using Cubic SVM, which shows the perfection of the proposed framework..Keywords
Cite This Article
Citations
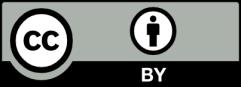
This work is licensed under a Creative Commons Attribution 4.0 International License , which permits unrestricted use, distribution, and reproduction in any medium, provided the original work is properly cited.