Open Access
ARTICLE
Bayesian Rule Modeling for Interpretable Mortality Classification of COVID-19 Patients
Software Department, Gachon University, Seongnam, 13120, Korea
* Corresponding Author: Myung-Mook Han. Email:
(This article belongs to the Special Issue: Artificial Intelligence and Healthcare Analytics for COVID-19)
Computers, Materials & Continua 2021, 69(3), 2827-2843. https://doi.org/10.32604/cmc.2021.017266
Received 25 January 2021; Accepted 25 March 2021; Issue published 24 August 2021
Abstract
Coronavirus disease 2019 (COVID-19) has been termed a “Pandemic Disease” that has infected many people and caused many deaths on a nearly unprecedented level. As more people are infected each day, it continues to pose a serious threat to humanity worldwide. As a result, healthcare systems around the world are facing a shortage of medical space such as wards and sickbeds. In most cases, healthy people experience tolerable symptoms if they are infected. However, in other cases, patients may suffer severe symptoms and require treatment in an intensive care unit. Thus, hospitals should select patients who have a high risk of death and treat them first. To solve this problem, a number of models have been developed for mortality prediction. However, they lack interpretability and generalization. To prepare a model that addresses these issues, we proposed a COVID-19 mortality prediction model that could provide new insights. We identified blood factors that could affect the prediction of COVID-19 mortality. In particular, we focused on dependency reduction using partial correlation and mutual information. Next, we used the Class-Attribute Interdependency Maximization (CAIM) algorithm to bin continuous values. Then, we used Jensen Shannon Divergence (JSD) and Bayesian posterior probability to create less redundant and more accurate rules. We provided a ruleset with its own posterior probability as a result. The extracted rules are in the form of “if antecedent then results, posterior probability()”. If the sample matches the extracted rules, then the result is positive. The average AUC Score was 96.77% for the validation dataset and the F1-score was 92.8% for the test data. Compared to the results of previous studies, it shows good performance in terms of classification performance, generalization, and interpretability.Keywords
Cite This Article
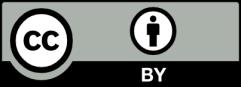