Open Access
ARTICLE
DeepIoT.IDS: Hybrid Deep Learning for Enhancing IoT Network Intrusion Detection
1 Faculty of Information and Communication Technology, Universiti Teknikal Malaysia, Melaka, 76100, Malaysia
2 Center of Intelligent and Autonomous Systems, Faculty of Computer Science and Information Technology, Universiti Tun Hussein Onn Malaysia, Johor, 86400, Malaysia
3 Faculty of Business and Technology, UNITAR International University, Selangor, 47301, Malaysia
4 School of Computing, Faculty of Engineering, Universiti Teknologi Malaysia, Johor, 81310, Malaysia
* Corresponding Author: Salama A. Mostafa. Email:
(This article belongs to the Special Issue: AI, IoT, Blockchain Assisted Intelligent Solutions to Medical and Healthcare Systems)
Computers, Materials & Continua 2021, 69(3), 3945-3966. https://doi.org/10.32604/cmc.2021.016074
Received 21 December 2020; Accepted 07 May 2021; Issue published 24 August 2021
Abstract
With an increasing number of services connected to the internet, including cloud computing and Internet of Things (IoT) systems, the prevention of cyberattacks has become more challenging due to the high dimensionality of the network traffic data and access points. Recently, researchers have suggested deep learning (DL) algorithms to define intrusion features through training empirical data and learning anomaly patterns of attacks. However, due to the high dynamics and imbalanced nature of the data, the existing DL classifiers are not completely effective at distinguishing between abnormal and normal behavior line connections for modern networks. Therefore, it is important to design a self-adaptive model for an intrusion detection system (IDS) to improve the detection of attacks. Consequently, in this paper, a novel hybrid weighted deep belief network (HW-DBN) algorithm is proposed for building an efficient and reliable IDS (DeepIoT.IDS) model to detect existing and novel cyberattacks. The HW-DBN algorithm integrates an improved Gaussian–Bernoulli restricted Boltzmann machine (Deep GB-RBM) feature learning operator with a weighted deep neural networks (WDNN) classifier. The CICIDS2017 dataset is selected to evaluate the DeepIoT.IDS model as it contains multiple types of attacks, complex data patterns, noise values, and imbalanced classes. We have compared the performance of the DeepIoT.IDS model with three recent models. The results show the DeepIoT.IDS model outperforms the three other models by achieving a higher detection accuracy of 99.38% and 99.99% for web attack and bot attack scenarios, respectively. Furthermore, it can detect the occurrence of low-frequency attacks that are undetectable by other models.Keywords
Cite This Article
Citations
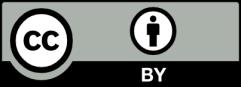
This work is licensed under a Creative Commons Attribution 4.0 International License , which permits unrestricted use, distribution, and reproduction in any medium, provided the original work is properly cited.