Open Access
ARTICLE
Addressing Economic Dispatch Problem with Multiple Fuels Using Oscillatory Particle Swarm Optimization
1 KIIT University, Bhubaneswar, Odisha, India
2 Department of Electrical Engineering, CET, Bhubaneswar, Odisha, India
3 Department of Computer Science, Government Bikram College of Commerce, Patiala, India
4 Department of Computer Science & Engineering, Pandit Deendayal Petroleum University, Gandhinagar, India
5 Raytheon Chair for Systems Engineering, Advanced Manufacturing Institute, King Saud University, Riyadh, 11421, Saudi Arabia
6 Industrial Engineering Department, College of Engineering, King Saud University, Riyadh, 11421, Saudi Arabia
* Corresponding Author: Mustufa Haider Abidi. Email:
(This article belongs to the Special Issue: Green IoT Networks using Machine Learning, Deep Learning Models)
Computers, Materials & Continua 2021, 69(3), 2863-2882. https://doi.org/10.32604/cmc.2021.016002
Received 17 December 2020; Accepted 20 February 2021; Issue published 24 August 2021
Abstract
Economic dispatch has a significant effect on optimal economical operation in the power systems in industrial revolution 4.0 in terms of considerable savings in revenue. Various non-linearity are added to make the fossil fuel-based power systems more practical. In order to achieve an accurate economical schedule, valve point loading effect, ramp rate constraints, and prohibited operating zones are being considered for realistic scenarios. In this paper, an improved, and modified version of conventional particle swarm optimization (PSO), called Oscillatory PSO (OPSO), is devised to provide a cheaper schedule with optimum cost. The conventional PSO is improved by deriving a mechanism enabling the particle towards the trajectories of oscillatory motion to acquire the entire search space. A set of differential equations is implemented to expose the condition for trajectory motion in oscillation. Using adaptive inertia weights, this OPSO method provides an optimized cost of generation as compared to the conventional particle swarm optimization and other new meta-heuristic approaches.Keywords
Cite This Article
Citations
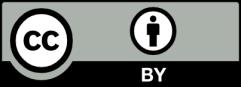
This work is licensed under a Creative Commons Attribution 4.0 International License , which permits unrestricted use, distribution, and reproduction in any medium, provided the original work is properly cited.