Open Access
ARTICLE
An Optimized Convolutional Neural Network Architecture Based on Evolutionary Ensemble Learning
1 College of Physical Education and Sports Sciences, University of Kirkuk, Kirkuk, 36001, Iraq
2 College of Administration & Economics, University of Kirkuk, Kirkuk, 36001, Iraq
3 Department of Information Technology, Institute of Graduate Studies and Research, Alexandria University, Alexandria, Egypt
4 Department of Computer Engineering, Alexandria Higher Institute of Engineering & Technology (AIET), Alexandria, Egypt
* Corresponding Author: Saad Darwish. Email:
(This article belongs to the Special Issue: Machine Learning-based Intelligent Systems: Theories, Algorithms, and Applications)
Computers, Materials & Continua 2021, 69(3), 3813-3828. https://doi.org/10.32604/cmc.2021.014759
Received 14 October 2020; Accepted 15 April 2021; Issue published 24 August 2021
Abstract
Convolutional Neural Networks (CNNs) models succeed in vast domains. CNNs are available in a variety of topologies and sizes. The challenge in this area is to develop the optimal CNN architecture for a particular issue in order to achieve high results by using minimal computational resources to train the architecture. Our proposed framework to automated design is aimed at resolving this problem. The proposed framework is focused on a genetic algorithm that develops a population of CNN models in order to find the architecture that is the best fit. In comparison to the co-authored work, our proposed framework is concerned with creating lightweight architectures with a limited number of parameters while retaining a high degree of validity accuracy utilizing an ensemble learning technique. This architecture is intended to operate on low-resource machines, rendering it ideal for implementation in a number of environments. Four common benchmark image datasets are used to test the proposed framework, and it is compared to peer competitors’ work utilizing a range of parameters, including accuracy, the number of model parameters used, the number of GPUs used, and the number of GPU days needed to complete the method. Our experimental findings demonstrated a significant advantage in terms of GPU days, accuracy, and the number of parameters in the discovered model.Keywords
Cite This Article
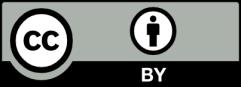