Open Access
ARTICLE
Generating Cartoon Images from Face Photos with Cycle-Consistent Adversarial Networks
1 School of Artificial Intelligence and Computer Science, Jiangnan University, Wuxi, 214000, China
2 Key Laboratory of Artificial Intelligence, Jiangsu, 214000, China
3 The Global Big Data Technologies Centre, University of Technology Sydney, Ultimo, NSW, 2007, Australia
4 The Institute of Image Processing and Pattern Recognition, Shanghai Jiao Tong University, Shanghai, 201100, China
* Corresponding Author: Zhanjie Zhang. Email:
Computers, Materials & Continua 2021, 69(2), 2733-2747. https://doi.org/10.32604/cmc.2021.019305
Received 08 April 2021; Accepted 09 May 2021; Issue published 21 July 2021
Abstract
The generative adversarial network (GAN) is first proposed in 2014, and this kind of network model is machine learning systems that can learn to measure a given distribution of data, one of the most important applications is style transfer. Style transfer is a class of vision and graphics problems where the goal is to learn the mapping between an input image and an output image. CYCLE-GAN is a classic GAN model, which has a wide range of scenarios in style transfer. Considering its unsupervised learning characteristics, the mapping is easy to be learned between an input image and an output image. However, it is difficult for CYCLE-GAN to converge and generate high-quality images. In order to solve this problem, spectral normalization is introduced into each convolutional kernel of the discriminator. Every convolutional kernel reaches Lipschitz stability constraint with adding spectral normalization and the value of the convolutional kernel is limited to [0, 1], which promotes the training process of the proposed model. Besides, we use pretrained model (VGG16) to control the loss of image content in the position of l1 regularization. To avoid overfitting, l1 regularization term and l2 regularization term are both used in the object loss function. In terms of Frechet Inception Distance (FID) score evaluation, our proposed model achieves outstanding performance and preserves more discriminative features. Experimental results show that the proposed model converges faster and achieves better FID scores than the state of the art.Keywords
Cite This Article
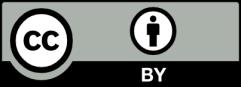
This work is licensed under a Creative Commons Attribution 4.0 International License , which permits unrestricted use, distribution, and reproduction in any medium, provided the original work is properly cited.