Open Access
ARTICLE
Lightweight Transfer Learning Models for Ultrasound-Guided Classification of COVID-19 Patients
1 College of Computing and Information Technology, Shaqra University, Shaqra, 11961, Saudi Arabia
2 Department of Industrial Electronics and Control Engineering, Faculty of Electronic Engineering (FEE), Menoufia University, Menouf, 32952, Egypt
3 Department of Mathematics and Computer Science, Faculty of Science, Sohag University, Sohag, 82524, Egypt
4 Department of Computer Engineering, College of Computers and Information Technology, Taif University, Taif, 21944, Saudi Arabia
5 Department of Physics, College of Sciences, University of Bisha, Bisha, 61922, Saudi Arabia
6 Department of Physics, Faculty of Science, Al-Azhar University, Assiut, 71524, Egypt
7 Department of Computer Science and Engineering, Faculty of Electronic Engineering (FEE), Menoufia University, Menouf, 32952, Egypt
* Corresponding Author: Marwa Ahmed Shouman. Email:
(This article belongs to the Special Issue: Role of Computer in Modelling & Solving Real-World Problems)
Computers, Materials & Continua 2021, 69(2), 2295-2312. https://doi.org/10.32604/cmc.2021.018671
Received 16 March 2021; Accepted 26 April 2021; Issue published 21 July 2021
Abstract
Lightweight deep convolutional neural networks (CNNs) present a good solution to achieve fast and accurate image-guided diagnostic procedures of COVID-19 patients. Recently, advantages of portable Ultrasound (US) imaging such as simplicity and safe procedures have attracted many radiologists for scanning suspected COVID-19 cases. In this paper, a new framework of lightweight deep learning classifiers, namely COVID-LWNet is proposed to identify COVID-19 and pneumonia abnormalities in US images. Compared to traditional deep learning models, lightweight CNNs showed significant performance of real-time vision applications by using mobile devices with limited hardware resources. Four main lightweight deep learning models, namely MobileNets, ShuffleNets, MENet and MnasNet have been proposed to identify the health status of lungs using US images. Public image dataset (POCUS) was used to validate our proposed COVID-LWNet framework successfully. Three classes of infectious COVID-19, bacterial pneumonia, and the healthy lung were investigated in this study. The results showed that the performance of our proposed MnasNet classifier achieved the best accuracy score and shortest training time of 99.0% and 647.0 s, respectively. This paper demonstrates the feasibility of using our proposed COVID-LWNet framework as a new mobile-based radiological tool for clinical diagnosis of COVID-19 and other lung diseases.Keywords
Cite This Article
Citations
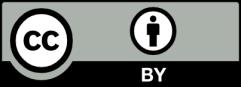
This work is licensed under a Creative Commons Attribution 4.0 International License , which permits unrestricted use, distribution, and reproduction in any medium, provided the original work is properly cited.