Open Access
ARTICLE
Towards Machine Learning Based Intrusion Detection in IoT Networks
1 Institute of Information Technology, Jahangirnagar University, Dhaka, Bangladesh
2 Department of Computer Science, Nottingham Trent University, Nottingham, UK
3 Division of Computer Science and Engineering, Jeonbuk National University, Jeonju, 54896, Korea
* Corresponding Author: Gi Hwan Cho. Email:
(This article belongs to the Special Issue: Emerging Applications of Artificial Intelligence, Machine learning and Data Science)
Computers, Materials & Continua 2021, 69(2), 1801-1821. https://doi.org/10.32604/cmc.2021.018466
Received 08 March 2021; Accepted 09 April 2021; Issue published 21 July 2021
Abstract
The Internet of Things (IoT) integrates billions of self-organized and heterogeneous smart nodes that communicate with each other without human intervention. In recent years, IoT based systems have been used in improving the experience in many applications including healthcare, agriculture, supply chain, education, transportation and traffic monitoring, utility services etc. However, node heterogeneity raised security concern which is one of the most complicated issues on the IoT. Implementing security measures, including encryption, access control, and authentication for the IoT devices are ineffective in achieving security. In this paper, we identified various types of IoT threats and shallow (such as decision tree (DT), random forest (RF), support vector machine (SVM)) as well as deep machine learning (deep neural network (DNN), deep belief network (DBN), long short-term memory (LSTM), stacked LSTM, bidirectional LSTM (Bi-LSTM)) based intrusion detection systems (IDS) in the IoT environment have been discussed. The performance of these models has been evaluated using five benchmark datasets such as NSL-KDD, IoTDevNet, DS2OS, IoTID20, and IoT Botnet dataset. The various performance metrics such as Accuracy, Precision, Recall, F1-score were used to evaluate the performance of shallow/deep machine learning based IDS. It has been found that deep machine learning IDS outperforms shallow machine learning in detecting IoT attacks.Keywords
Cite This Article
Citations
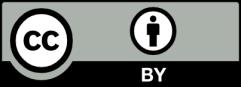
This work is licensed under a Creative Commons Attribution 4.0 International License , which permits unrestricted use, distribution, and reproduction in any medium, provided the original work is properly cited.