Open Access
ARTICLE
Toward Robust Classifiers for PDF Malware Detection
College of Computers in Al-Leith, Umm Al Qura University, Makkah, Saudi Arabia
* Corresponding Author: Marwan Albahar. Email:
Computers, Materials & Continua 2021, 69(2), 2181-2202. https://doi.org/10.32604/cmc.2021.018260
Received 03 March 2021; Accepted 19 April 2021; Issue published 21 July 2021
Abstract
Malicious Portable Document Format (PDF) files represent one of the largest threats in the computer security space. Significant research has been done using handwritten signatures and machine learning based on detection via manual feature extraction. These approaches are time consuming, require substantial prior knowledge, and the list of features must be updated with each newly discovered vulnerability individually. In this study, we propose two models for PDF malware detection. The first model is a convolutional neural network (CNN) integrated into a standard deviation based regularization model to detect malicious PDF documents. The second model is a support vector machine (SVM) based ensemble model with three different kernels. The two models were trained and tested on two different datasets. The experimental results show that the accuracy of both models is approximately 100%, and the robustness against evasive samples is excellent. Further, the robustness of the models was evaluated with malicious PDF documents generated using Mimicus. Both models can distinguish the different vulnerabilities exploited in malicious files and achieve excellent performance in terms of generalization ability, accuracy, and robustness.Keywords
Cite This Article
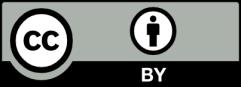
This work is licensed under a Creative Commons Attribution 4.0 International License , which permits unrestricted use, distribution, and reproduction in any medium, provided the original work is properly cited.