Open Access
ARTICLE
A Novel AlphaSRGAN for Underwater Image Super Resolution
SRM Institute of Science and Technology, Chennai, India
* Corresponding Author: Aswathy K. Cherian. Email:
(This article belongs to the Special Issue: Computational Models for Pro-Smart Environments in Data Science Assisted IoT Systems)
Computers, Materials & Continua 2021, 69(2), 1537-1552. https://doi.org/10.32604/cmc.2021.018213
Received 01 March 2021; Accepted 02 April 2021; Issue published 21 July 2021
Abstract
Obtaining clear images of underwater scenes with descriptive details is an arduous task. Conventional imaging techniques fail to provide clear cut features and attributes that ultimately result in object recognition errors. Consequently, a need for a system that produces clear images for underwater image study has been necessitated. To overcome problems in resolution and to make better use of the Super-Resolution (SR) method, this paper introduces a novel method that has been derived from the Alpha Generative Adversarial Network (AlphaGAN) model, named Alpha Super Resolution Generative Adversarial Network (AlphaSRGAN). The model put forth in this paper helps in enhancing the quality of underwater imagery and yields images with greater resolution and more concise details. Images undergo pre-processing before they are fed into a generator network that optimizes and reforms the structure of the network while enhancing the stability of the network that acts as the generator. After the images are processed by the generator network, they are passed through an adversarial method for training models. The dataset used in this paper to learn Single Image Super Resolution (SISR) is the USR 248 dataset. Training supervision is performed by an unprejudiced function that simultaneously scrutinizes and improves the image quality. Appraisal of images is done with reference to factors like local style information, global content and color. The dataset USR 248 which has a huge collection of images has been used for the study is composed of three collections of images—high (640 480) and low (80 60, 160 120, and 320 240). Paired instances of different sizes—2, 4 and 8—are also present in the dataset. Parameters like Mean Opinion Score (MOS), Peak Signal-to-Noise Ratio (PSNR), Structural Similarity (SSIM) and Underwater Image Quality Measure (UIQM) scores have been compared to validate the improved efficiency of our model when compared to existing works.Keywords
Cite This Article
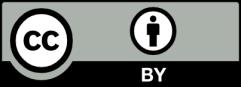
This work is licensed under a Creative Commons Attribution 4.0 International License , which permits unrestricted use, distribution, and reproduction in any medium, provided the original work is properly cited.