Open Access
ARTICLE
Enhanced Fingerprinting Based Indoor Positioning Using Machine Learning
1 Department of Electrical Engineering, National University of Sciences and Technology, Islamabad, 44000, Pakistan
2 Department of Computer Engineering, National University of Modern Languages (NUML), Islamabad, 44000, Pakistan
3 Faculty of Computing, Capital University of Science and Technology, Islamabad, 44000, Pakistan
* Corresponding Author: Alina Mirza. Email:
(This article belongs to the Special Issue: Reinforcement Learning Based solutions for Next-Generation Wireless Networks Coexistence)
Computers, Materials & Continua 2021, 69(2), 1631-1652. https://doi.org/10.32604/cmc.2021.018205
Received 01 March 2021; Accepted 05 April 2021; Issue published 21 July 2021
Abstract
Due to the inability of the Global Positioning System (GPS) signals to penetrate through surfaces like roofs, walls, and other objects in indoor environments, numerous alternative methods for user positioning have been presented. Amongst those, the Wi-Fi fingerprinting method has gained considerable interest in Indoor Positioning Systems (IPS) as the need for line-of-sight measurements is minimal, and it achieves better efficiency in even complex indoor environments. Offline and online are the two phases of the fingerprinting method. Many researchers have highlighted the problems in the offline phase as it deals with huge datasets and validation of Fingerprints without pre-processing of data becomes a concern. Machine learning is used for the model training in the offline phase while the locations are estimated in the online phase. Many researchers have considered the concerns in the offline phase as it deals with huge datasets and validation of Fingerprints becomes an issue. Machine learning algorithms are a natural solution for winnowing through large datasets and determining the significant fragments of information for localization, creating precise models to predict an indoor location. Large training sets are a key for obtaining better results in machine learning problems. Therefore, an existing WLAN fingerprinting-based multi-story building location database has been used with 21049 samples including 19938 training and 1111 testing samples. The proposed model consists of mean and median filtering as pre-processing techniques applied to the database for enhancing the accuracy by mitigating the impact of environmental dispersion and investigated machine learning algorithms (kNN, WkNN, FSkNN, and SVM) for estimating the location. The proposed SVM with median filtering algorithm gives a reduced mean positioning error of 0.7959 m and an improved efficiency of 92.84% as compared to all variants of the proposed method for 108703 m2 area.Keywords
Cite This Article
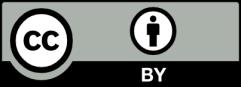