Open Access
ARTICLE
Predicting the Need for ICU Admission in COVID-19 Patients Using XGBoost
1 College of Computer and Information Sciences, Jouf University, Sakaka, KSA
2 Faculty of Engineering, Al Azhar University, Cairo, Egypt
3 Faculty of Mathematical and Computer Sciences, University of Gezira, Wad Madani, Sudan
4 Faculty of Medicine, Al Azhar University, Cairo, Egypt
5 College of Medicine, Shaqra University, KSA
* Corresponding Author: Mohamed Ezz. Email:
(This article belongs to the Special Issue: Machine Learning-based Intelligent Systems: Theories, Algorithms, and Applications)
Computers, Materials & Continua 2021, 69(2), 2077-2092. https://doi.org/10.32604/cmc.2021.018155
Received 27 February 2021; Accepted 19 April 2021; Issue published 21 July 2021
Abstract
It is important to determine early on which patients require ICU admissions in managing COVID-19 especially when medical resources are limited. Delay in ICU admissions is associated with negative outcomes such as mortality and cost. Therefore, early identification of patients with a high risk of respiratory failure can prevent complications, enhance risk stratification, and improve the outcomes of severely-ill hospitalized patients. In this paper, we develop a model that uses the characteristics and information collected at the time of patients’ admissions and during their early period of hospitalization to accurately predict whether they will need ICU admissions. We use the data explained and organized in a window-based manner by the Sírio-Libanês hospital team (published on Kaggle). Preprocessing is applied, including imputation, cleaning, and feature selection. In the cleaning process, we remove zero-variance, redundant, and/or highly correlated (measured by the Pearson correlation coefficient) features. We use Extreme Gradient Boosting (XGBoost) with early stopping as a predictor in our developed model. We run the experiment in four stages starting from the features of Window 1 in Stage 1 and then incrementally add the features of Windows 2–4 in Stages 2–4 respectively. We achieve AUCs of 0.73, 0.92, 0.95, and 0.97 in those four stages.Keywords
Cite This Article
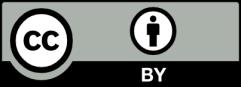
This work is licensed under a Creative Commons Attribution 4.0 International License , which permits unrestricted use, distribution, and reproduction in any medium, provided the original work is properly cited.