Open Access
ARTICLE
Real-Time Violent Action Recognition Using Key Frames Extraction and Deep Learning
1 Deparment of Computer Science and Information Technology, The University of Lahore, Sargodha Campus, Sargodha, 40100, Pakistan
2 Department of Computer Science, COMSATS University Islamabad, Wah Campus, Wah Cantt, 47040, Pakistan
3 School of System and Technology, University of Management and Technology, Lahore, 54782, Pakistan
4 Department of Computer Science and Information Technology, University of Sargodha, Sargodha, 40100, Pakistan
5 College of Engineering, Al Ain University, Al Ain, United Arab Emirates
6 Department of Computer Science, HITEC University Taxila, Taxila, Pakistan
7 Applied Artificial Intelligence, Ajou University, Suwon, Korea
8 Department of Computer Science and Engineering, Soonchunhyang University, Asan, Korea
9 Department of Mathematics and Computer Science, Faculty of Science, Beirut Arab University, Lebanon
* Corresponding Author: Yunyoung Nam. Email:
Computers, Materials & Continua 2021, 69(2), 2217-2230. https://doi.org/10.32604/cmc.2021.018103
Received 24 February 2021; Accepted 24 April 2021; Issue published 21 July 2021
Abstract
Violence recognition is crucial because of its applications in activities related to security and law enforcement. Existing semi-automated systems have issues such as tedious manual surveillances, which causes human errors and makes these systems less effective. Several approaches have been proposed using trajectory-based, non-object-centric, and deep-learning-based methods. Previous studies have shown that deep learning techniques attain higher accuracy and lower error rates than those of other methods. However, the their performance must be improved. This study explores the state-of-the-art deep learning architecture of convolutional neural networks (CNNs) and inception V4 to detect and recognize violence using video data. In the proposed framework, the keyframe extraction technique eliminates duplicate consecutive frames. This keyframing phase reduces the training data size and hence decreases the computational cost by avoiding duplicate frames. For feature selection and classification tasks, the applied sequential CNN uses one kernel size, whereas the inception v4 CNN uses multiple kernels for different layers of the architecture. For empirical analysis, four widely used standard datasets are used with diverse activities. The results confirm that the proposed approach attains 98% accuracy, reduces the computational cost, and outperforms the existing techniques of violence detection and recognition.Keywords
Cite This Article
Citations
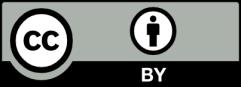
This work is licensed under a Creative Commons Attribution 4.0 International License , which permits unrestricted use, distribution, and reproduction in any medium, provided the original work is properly cited.