Open Access
ARTICLE
Automated Disassembly Sequence Prediction for Industry 4.0 Using Enhanced Genetic Algorithm
1 Department of Mechanical Engineering, Industrial Robotics and Manufacturing Automation Laboratory, National Institute of Technology, Kariakal, 609609, India
2 Department of Computer Science Engineering, National Institute of Technology, Kariakal, 609609, India
3 Raytheon Chair for Systems Engineering, Advanced Manufacturing Institute, King Saud University, Riyadh, 11421, Saudi Arabia
4 Industrial Engineering Department, College of Engineering, King Saud University, Riyadh, 11421, Saudi Arabia
* Corresponding Author: Mustufa Haider Abidi. Email:
(This article belongs to the Special Issue: Emerging Trends in Software-Defined Networking for Industry 4.0)
Computers, Materials & Continua 2021, 69(2), 2531-2548. https://doi.org/10.32604/cmc.2021.018014
Received 21 February 2021; Accepted 17 April 2021; Issue published 21 July 2021
Abstract
The evolution of Industry 4.0 made it essential to adopt the Internet of Things (IoT) and Cloud Computing (CC) technologies to perform activities in the new age of manufacturing. These technologies enable collecting, storing, and retrieving essential information from the manufacturing stage. Data collected at sites are shared with others where execution automatedly occurs. The obtained information must be validated at manufacturing to avoid undesirable data losses during the de-manufacturing process. However, information sharing from the assembly level at the manufacturing stage to disassembly at the product end-of-life state is a major concern. The current research validates the information optimally to offer a minimum set of activities to complete the disassembly process. An optimal disassembly sequence plan (DSP) can possess valid information to organize the necessary actions in manufacturing. However, finding an optimal DSP is complex because of its combinatorial nature. The genetic algorithm (GA) is a widely preferred artificial intelligence (AI) algorithm to obtain a near-optimal solution for the DSP problem. The converging nature at local optima is a limitation in the traditional GA. This study improvised the GA workability by integrating with the proposed priori crossover operator. An optimality function is defined to reduce disassembly effort by considering directional changes as parameters. The enhanced GA method is tested on a real-time product to evaluate the performance. The obtained results reveal that diversity control depends on the operators employed in the disassembly attributes. The proposed method’s solution can be stored in the cloud and shared through IoT devices for effective resource allocation and disassembly for maximum recovery of the product. The effectiveness of the proposed enhanced GA method is determined by making a comparative assessment with traditional GA and other AI methods at different population sizes.Keywords
Cite This Article
Citations
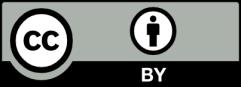