Open Access
ARTICLE
A Novel Framework for Multi-Classification of Guava Disease
1 College of Applied Computer Sciences, King Saud University (Almuzahmiyah Branch), Riyadh, 11564, KSA
2 Department of Computer Science, University of Central Punjab, Lahore, 54000, Pakistan
3 Department of CS&SE, International Islamic University, Islamabad, 44000, Pakistan
4 Division of Science and Technology, University of Education, Lahore, 54000, Pakistan
5 Department of Electrical and Computer Engineering, Dhofar University, Salalah, Oman
* Corresponding Author: Awais Mahmood. Email:
(This article belongs to the Special Issue: Artificial Intelligence based Smart precision agriculture with analytic pattern in sustainable environments using IoT)
Computers, Materials & Continua 2021, 69(2), 1915-1926. https://doi.org/10.32604/cmc.2021.017702
Received 08 February 2021; Accepted 19 April 2021; Issue published 21 July 2021
Abstract
Guava is one of the most important fruits in Pakistan, and is gradually boosting the economy of Pakistan. Guava production can be interrupted due to different diseases, such as anthracnose, algal spot, fruit fly, styler end rot and canker. These diseases are usually detected and identified by visual observation, thus automatic detection is required to assist formers. In this research, a new technique was created to detect guava plant diseases using image processing techniques and computer vision. An automated system is developed to support farmers to identify major diseases in guava. We collected healthy and unhealthy images of different guava diseases from the field. Then image labeling was done with the help of an expert to differentiate between healthy and unhealthy fruit. The local binary pattern (LBP) was used for the extraction of features, and principal component analysis (PCA) was used for dimensionality reduction. Disease classification was carried out using multiple classifiers, including cubic support vector machine, Fine K-nearest neighbor (F-KNN), Bagged Tree and RUSBoosted Tree algorithms and achieved 100% accuracy for the diagnosis of fruit flies disease using Bagged Tree. However, the findings indicated that cubic support vector machines (C-SVM) was the best classifier for all guava disease mentioned in the dataset.Keywords
Cite This Article
Citations
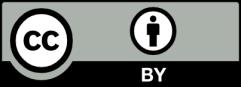