Open Access
ARTICLE
Conveyor-Belt Detection of Conditional Deep Convolutional Generative Adversarial Network
1 College of Information and Computer, Taiyuan University of Technology, Jinzhong, Shanxi, 030600, China
2 State Grid Taiyuan Power Supply Company, Taiyuan, Shanxi, 030012, China
3 International Business Machines Corporation (IBM), NY, USA
* Corresponding Author: Xiaoli Hao. Email:
Computers, Materials & Continua 2021, 69(2), 2671-2685. https://doi.org/10.32604/cmc.2021.016856
Received 13 January 2021; Accepted 19 March 2021; Issue published 21 July 2021
Abstract
In underground mining, the belt is a critical component, as its state directly affects the safe and stable operation of the conveyor. Most of the existing non-contact detection methods based on machine vision can only detect a single type of damage and they require pre-processing operations. This tends to cause a large amount of calculation and low detection precision. To solve these problems, in the work described in this paper a belt tear detection method based on a multi-class conditional deep convolutional generative adversarial network (CDCGAN) was designed. In the traditional DCGAN, the image generated by the generator has a certain degree of randomness. Here, a small number of labeled belt images are taken as conditions and added them to the generator and discriminator, so the generator can generate images with the characteristics of belt damage under the aforementioned conditions. Moreover, because the discriminator cannot identify multiple types of damage, the multi-class softmax function is used as the output function of the discriminator to output a vector of class probabilities, and it can accurately classify cracks, scratches, and tears. To avoid the features learned incompletely, skip-layer connection is adopted in the generator and discriminator. This not only can minimize the loss of features, but also improves the convergence speed. Compared with other algorithms, experimental results show that the loss value of the generator and discriminator is the least. Moreover, its convergence speed is faster, and the mean average precision of the proposed algorithm is up to 96.2%, which is at least 6% higher than that of other algorithms.Keywords
Cite This Article
Citations
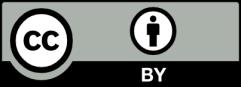
This work is licensed under a Creative Commons Attribution 4.0 International License , which permits unrestricted use, distribution, and reproduction in any medium, provided the original work is properly cited.