Open Access
ARTICLE
An Adaptive Lasso Grey Model for Regional FDI Statistics Prediction
1 Centre for Innovation Research in Social Governance, Changsha University of Science and Technology, Changsha, 410114, China
2 College of Information Technology and Management, Hunan University of Finance and Economics, Changsha, 410205, China
3 Department of Mathematics and Computer Science, Northeastern State University, Tahlequah, 74464, USA
* Corresponding Author: Huajun Huang. Email:
Computers, Materials & Continua 2021, 69(2), 2111-2121. https://doi.org/10.32604/cmc.2021.016770
Received 11 January 2021; Accepted 26 April 2021; Issue published 21 July 2021
Abstract
To overcome the deficiency of traditional mathematical statistics methods, an adaptive Lasso grey model algorithm for regional FDI (foreign direct investment) prediction is proposed in this paper, and its validity is analyzed. Firstly, the characteristics of the FDI data in six provinces of Central China are generalized, and the mixture model's constituent variables of the Lasso grey problem as well as the grey model are defined. Next, based on the influencing factors of regional FDI statistics (mean values of regional FDI and median values of regional FDI), an adaptive Lasso grey model algorithm for regional FDI was established. Then, an application test in Central China is taken as a case study to illustrate the feasibility of the adaptive Lasso grey model algorithm in regional FDI prediction. We also select RMSE (root mean square error) and MAE (mean absolute error) to demonstrate the convergence and the validity of the algorithm. Finally, we train this proposedal gorithm according to the regional FDI statistical data in six provinces in Central China from 2006 to 2018. We then use it to predict the regional FDI statistical data from 2019 to 2023 and show its changing tendency. The extended work for the adaptive Lasso grey model algorithm and its procedure to other regional economic fields is also discussed.Keywords
Cite This Article
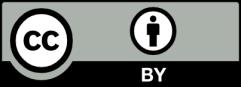
This work is licensed under a Creative Commons Attribution 4.0 International License , which permits unrestricted use, distribution, and reproduction in any medium, provided the original work is properly cited.