Open Access
ARTICLE
Adapted Long Short-Term Memory (LSTM) for Concurrent\\ Human Activity Recognition
Department of Electronic Engineering, Kwangwoon University, Seoul, 139701, Korea
* Corresponding Author: Yang Sung-Hyun. Email:
Computers, Materials & Continua 2021, 69(2), 1653-1670. https://doi.org/10.32604/cmc.2021.015660
Received 01 December 2020; Accepted 14 April 2021; Issue published 21 July 2021
Abstract
In this era, deep learning methods offer a broad spectrum of efficient and original algorithms to recognize or predict an output when given a sequence of inputs. In current trends, deep learning methods using recent long short-term memory (LSTM) algorithms try to provide superior performance, but they still have limited effectiveness when detecting sequences of complex human activity. In this work, we adapted the LSTM algorithm into a synchronous algorithm (sync-LSTM), enabling the model to take multiple parallel input sequences to produce multiple parallel synchronized output sequences. The proposed method is implemented for simultaneous human activity recognition (HAR) using heterogeneous sensor data in a smart home. HAR assists artificial intelligence in providing services to users according to their preferences. The sync-LSTM algorithm improves learning performance and sees its potential for real-world applications in complex HAR, such as concurrent activity, with higher accuracy and satisfactory computational complexity. The adapted algorithm for HAR is also applicable in the fields of ambient assistive living, healthcare, robotics, pervasive computing, and astronomy. Extensive experimental evaluation with publicly available datasets demonstrates the competitive recognition capabilities of our approach. The sync-LSTM algorithm improves learning performance and has the potential for real-life applications in complex HAR. For concurrent activity recognition, our proposed method shows an accuracy of more than 97%.Keywords
Cite This Article
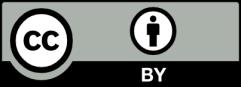
This work is licensed under a Creative Commons Attribution 4.0 International License , which permits unrestricted use, distribution, and reproduction in any medium, provided the original work is properly cited.