Open Access
ARTICLE
Classification of Epileptic Electroencephalograms Using Time-Frequency and Back Propagation Methods
1 Department of Computer Engineering, Halic University, Istanbul, 34445, Turkey
2 Department of Computer Engineering, İstanbul University—Cerrahpasa, Istanbul, 34320, Turkey
3 Department of Computer Engineering, Sivas Cumhuriyet University, Sivas, 58140, Turkey
* Corresponding Author: Sengul Bayrak. Email:
(This article belongs to the Special Issue: Intelligent Decision Support Systems for Complex Healthcare Applications)
Computers, Materials & Continua 2021, 69(2), 1427-1446. https://doi.org/10.32604/cmc.2021.015524
Received 25 November 2020; Accepted 20 January 2021; Issue published 21 July 2021
Abstract
Today, electroencephalography is used to measure brain activity by creating signals that are viewed on a monitor. These signals are frequently used to obtain information about brain neurons and may detect disorders that affect the brain, such as epilepsy. Electroencephalogram (EEG) signals are however prone to artefacts. These artefacts must be removed to obtain accurate and meaningful signals. Currently, computer-aided systems have been used for this purpose. These systems provide high computing power, problem-specific development, and other advantages. In this study, a new clinical decision support system was developed for individuals to detect epileptic seizures using EEG signals. Comprehensive classification results were obtained for the extracted filtered features from the time-frequency domain. The classification accuracies of the time-frequency features obtained from discrete continuous transform (DCT), fractional Fourier transform (FrFT), and Hilbert transform (HT) are compared. Artificial neural networks (ANN) were applied, and back propagation (BP) was used as a learning method. Many studies in the literature describe a single BP algorithm. In contrast, we looked at several BP algorithms including gradient descent with momentum (GDM), scaled conjugate gradient (SCG), and gradient descent with adaptive learning rate (GDA). The most successful algorithm was tested using simulations made on three separate datasets (DCT_EEG, FrFT_EEG, and HT_EEG) that make up the input data. The HT algorithm was the most successful EEG feature extractor in terms of classification accuracy rates in each EEG dataset and had the highest referred accuracy rates of the algorithms. As a result, HT_EEG gives the highest accuracy for all algorithms, and the highest accuracy of 87.38% was produced by the SCG algorithm.Keywords
Cite This Article
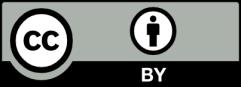