Open Access
ARTICLE
Few-Shot Learning for Discovering Anomalous Behaviors in Edge Networks
1 Department of Information Technology, Helwan University, Cairo, Egypt
2 Department of Computer Science, Helwan University, Cairo, Egypt
3 School of Engineering and Information Technology, University of New South Wales at ADFA, Canberra, Australia
* Corresponding Author: Nour Moustafa. Email:
(This article belongs to the Special Issue: Security and Computing in Internet of Things)
Computers, Materials & Continua 2021, 69(2), 1823-1837. https://doi.org/10.32604/cmc.2021.012877
Received 30 August 2020; Accepted 16 April 2021; Issue published 21 July 2021
Abstract
Intrusion Detection Systems (IDSs) have a great interest these days to discover complex attack events and protect the critical infrastructures of the Internet of Things (IoT) networks. Existing IDSs based on shallow and deep network architectures demand high computational resources and high volumes of data to establish an adaptive detection engine that discovers new families of attacks from the edge of IoT networks. However, attackers exploit network gateways at the edge using new attacking scenarios (i.e., zero-day attacks), such as ransomware and Distributed Denial of Service (DDoS) attacks. This paper proposes new IDS based on Few-Shot Deep Learning, named CNN-IDS, which can automatically identify zero-day attacks from the edge of a network and protect its IoT systems. The proposed system comprises two-methodological stages: 1) a filtered Information Gain method is to select the most useful features from network data, and 2) one-dimensional Convolutional Neural Network (CNN) algorithm is to recognize new attack types from a network's edge. The proposed model is trained and validated using two datasets of the UNSW-NB15 and Bot-IoT. The experimental results showed that it enhances about a 3% detection rate and around a 3%–4% false-positive rate with the UNSW-NB15 dataset and about an 8% detection rate using the BoT-IoT dataset.Keywords
Cite This Article
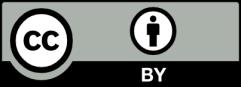