Open Access
ARTICLE
Intelligent Multiclass Skin Cancer Detection Using Convolution Neural Networks
Department of Information Technology, College of Computer and Information Sciences, King Saud University, Riyadh, Saudi Arabia
* Corresponding Author: Reham Alabduljabbar. Email:
(This article belongs to the Special Issue: Emerging Trends in Artificial Intelligence and Machine Learning)
Computers, Materials & Continua 2021, 69(1), 831-847. https://doi.org/10.32604/cmc.2021.018402
Received 05 March 2021; Accepted 07 April 2021; Issue published 04 June 2021
Abstract
The worldwide mortality rate due to cancer is second only to cardiovascular diseases. The discovery of image processing, latest artificial intelligence techniques, and upcoming algorithms can be used to effectively diagnose and prognose cancer faster and reduce the mortality rate. Efficiently applying these latest techniques has increased the survival chances during recent years. The research community is making significant continuous progress in developing automated tools to assist dermatologists in decision making. The datasets used for the experimentation and analysis are ISBI 2016, ISBI 2017, and HAM 10000. In this work pertained models are used to extract the efficient feature. The pertained models applied are ResNet, InceptionV3, and classical feature extraction techniques. Before that, efficient preprocessing is conducted on dermoscopic images by applying various data augmentation techniques. Further, for classification, convolution neural networks were implemented. To classify dermoscopic images on HAM 1000 Dataset, the maximum attained accuracy is 89.30% for the proposed technique. The other parameters for measuring the performance attained 87.34% (Sen), 86.33% (Pre), 88.44% (F1-S), and 11.30% false-negative rate (FNR). The class with the highest TP rate is 97.6% for Melanoma; whereas, the lowest TP rate was for the Dermatofibroma class. For dataset ISBI2016, the accuracy achieved is 97.0% with the proposed classifier, whereas the other parameters for validation are 96.12% (Sen), 97.01% (Pre), 96.3% (F1-S), and further 3.7% (FNR). For the experiment with the ISBI2017 dataset, Sen, Pre, F1-S, and FNR were 93.9%, 94.9%, 93.9%, and 5.2%, respectively.Keywords
Cite This Article
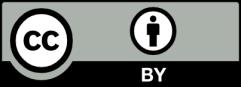
This work is licensed under a Creative Commons Attribution 4.0 International License , which permits unrestricted use, distribution, and reproduction in any medium, provided the original work is properly cited.