Open Access
ARTICLE
Deep Learning and Entity Embedding-Based Intrusion Detection Model for Wireless Sensor Networks
Department of Computer Science, College of Computer Engineering and Sciences, Prince Sattam bin Abdulaziz University, Al-Kharj, 11942, Saudi Arabia
* Corresponding Author: Bandar Almaslukh. Email:
Computers, Materials & Continua 2021, 69(1), 1343-1360. https://doi.org/10.32604/cmc.2021.017914
Received 17 February 2021; Accepted 11 April 2021; Issue published 04 June 2021
Abstract
Wireless sensor networks (WSNs) are considered promising for applications such as military surveillance and healthcare. The security of these networks must be ensured in order to have reliable applications. Securing such networks requires more attention, as they typically implement no dedicated security appliance. In addition, the sensors have limited computing resources and power and storage, which makes WSNs vulnerable to various attacks, especially denial of service (DoS). The main types of DoS attacks against WSNs are blackhole, grayhole, flooding, and scheduling. There are two primary techniques to build an intrusion detection system (IDS): signature-based and data-driven-based. This study uses the data-driven approach since the signature-based method fails to detect a zero-day attack. Several publications have proposed data-driven approaches to protect WSNs against such attacks. These approaches are based on either the traditional machine learning (ML) method or a deep learning model. The fundamental limitations of these methods include the use of raw features to build an intrusion detection model, which can result in low detection accuracy. This study implements entity embedding to transform the raw features to a more robust representation that can enable more precise detection and demonstrates how the proposed method can outperform state-of-the-art solutions in terms of recognition accuracy.Keywords
Cite This Article
Citations
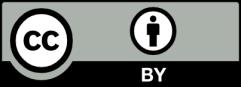
This work is licensed under a Creative Commons Attribution 4.0 International License , which permits unrestricted use, distribution, and reproduction in any medium, provided the original work is properly cited.