Open Access
ARTICLE
An Efficient CNN-Based Automated Diagnosis Framework from COVID-19 CT Images
1 Department Electronics and Electrical Communications, Faculty of Electronic Engineering, Menoufia University, Menouf, 32952, Egypt
2 Department of Electronics and Electrical Communications, Faculty of Engineering, Minia University, Egypt
3 Department of Industrial Electronics and Control Engineering, Faculty of Electronic Engineering, Menoufia University, Menouf, 32952, Egypt
4 Department of Information Technology, College of Computer and Information Sciences, Princess Nourah Bint Abdulrahman University, Riyadh, 84428, Saudi Arabia
* Corresponding Author: Naglaa F. Soliman. Email:
Computers, Materials & Continua 2021, 69(1), 1323-1341. https://doi.org/10.32604/cmc.2021.017385
Received 29 January 2021; Accepted 12 April 2021; Issue published 04 June 2021
Abstract
Corona Virus Disease-2019 (COVID-19) continues to spread rapidly in the world. It has dramatically affected daily lives, public health, and the world economy. This paper presents a segmentation and classification framework of COVID-19 images based on deep learning. Firstly, the classification process is employed to discriminate between COVID-19, non-COVID, and pneumonia by Convolutional Neural Network (CNN). Then, the segmentation process is applied for COVID-19 and pneumonia CT images. Finally, the resulting segmented images are used to identify the infected region, whether COVID-19 or pneumonia. The proposed CNN consists of four Convolutional (Conv) layers, four batch normalization layers, and four Rectified Linear Units (ReLUs). The sizes of Conv layer used filters are 8, 16, 32, and 64. Four max-pooling layers are employed with a stride of 2 and a 2 × 2 window. The classification layer comprises a Fully-Connected (FC) layer and a soft-max activation function used to take the classification decision. A novel saliency-based region detection algorithm and an active contour segmentation strategy are applied to segment COVID-19 and pneumonia CT images. The acquired findings substantiate the efficacy of the proposed framework for helping the specialists in automated diagnosis applications.Keywords
Cite This Article
Citations
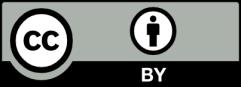