Open Access
ARTICLE
Guided Intra-Patch Smoothing Graph Filtering for Single-Image Denoising
1 College of Internet of Things Engineering, Hohai University, Changzhou, 213022, China
2 School of Microelectronics and Control Engineering, Changzhou University, Changzhou, 213022, China
3 Department of Electrical Engineering, University of Windsor, Ontario, N9B 3P4, Canada
* Corresponding Author: Yan Zhou. Email:
Computers, Materials & Continua 2021, 69(1), 67-80. https://doi.org/10.32604/cmc.2021.017300
Received 26 January 2021; Accepted 04 March 2021; Issue published 04 June 2021
Abstract
Graph filtering is an important part of graph signal processing and a useful tool for image denoising. Existing graph filtering methods, such as adaptive weighted graph filtering (AWGF), focus on coefficient shrinkage strategies in a graph-frequency domain. However, they seldom consider the image attributes in their graph-filtering procedure. Consequently, the denoising performance of graph filtering is barely comparable with that of other state-of-the-art denoising methods. To fully exploit the image attributes, we propose a guided intra-patch smoothing AWGF (AWGF-GPS) method for single-image denoising. Unlike AWGF, which employs graph topology on patches, AWGF-GPS learns the topology of superpixels by introducing the pixel smoothing attribute of a patch. This operation forces the restored pixels to smoothly evolve in local areas, where both intra- and inter-patch relationships of the image are utilized during patch restoration. Meanwhile, a guided-patch regularizer is incorporated into AWGF-GPS. The guided patch is obtained in advance using a maximum-a-posteriori probability estimator. Because the guided patch is considered as a sketch of a denoised patch, AWGF-GPS can effectively supervise patch restoration during graph filtering to increase the reliability of the denoised patch. Experiments demonstrate that the AWGF-GPS method suitably rebuilds denoising images. It outperforms most state-of-the-art single-image denoising methods and is competitive with certain deep-learning methods. In particular, it has the advantage of managing images with significant noise.Keywords
Cite This Article
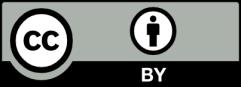
This work is licensed under a Creative Commons Attribution 4.0 International License , which permits unrestricted use, distribution, and reproduction in any medium, provided the original work is properly cited.