Open Access
ARTICLE
Segmentation and Classification of Stomach Abnormalities Using Deep Learning
1 Department of Computer Science, HITEC University Taxila, Taxila, Pakistan
2 College of Computer Science and Engineering, University of Ha’il, Ha’il, Saudi Arabia
3 Department of Software, Sejong University, Seoul, Korea, Gwangjin-gu, Korea
4 College of Computer Engineering and Sciences, Prince Sattam Bin Abdulaziz University, Al-Khraj, Saudi Arabia
5 Faculty of Applied Computing and Technology, Noroff University College, Kristiansand, Norway
* Corresponding Author: Oh-Young Song. Email:
(This article belongs to the Special Issue: Recent Advances in Deep Learning for Medical Image Analysis)
Computers, Materials & Continua 2021, 69(1), 607-625. https://doi.org/10.32604/cmc.2021.017101
Received 18 January 2021; Accepted 21 February 2021; Issue published 04 June 2021
Abstract
An automated system is proposed for the detection and classification of GI abnormalities. The proposed method operates under two pipeline procedures: (a) segmentation of the bleeding infection region and (b) classification of GI abnormalities by deep learning. The first bleeding region is segmented using a hybrid approach. The threshold is applied to each channel extracted from the original RGB image. Later, all channels are merged through mutual information and pixel-based techniques. As a result, the image is segmented. Texture and deep learning features are extracted in the proposed classification task. The transfer learning (TL) approach is used for the extraction of deep features. The Local Binary Pattern (LBP) method is used for texture features. Later, an entropy-based feature selection approach is implemented to select the best features of both deep learning and texture vectors. The selected optimal features are combined with a serial-based technique and the resulting vector is fed to the Ensemble Learning Classifier. The experimental process is evaluated on the basis of two datasets: Private and KVASIR. The accuracy achieved is 99.8 per cent for the private data set and 86.4 percent for the KVASIR data set. It can be confirmed that the proposed method is effective in detecting and classifying GI abnormalities and exceeds other methods of comparison.Keywords
Cite This Article
Citations
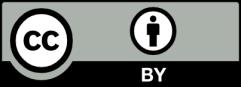
This work is licensed under a Creative Commons Attribution 4.0 International License , which permits unrestricted use, distribution, and reproduction in any medium, provided the original work is properly cited.