Open Access
ARTICLE
Machine Learning-Based Two-Stage Data Selection Scheme for Long-Term Influenza Forecasting
School of Electrical Engineering, Korea University, Seoul, 02841, Korea
* Corresponding Author: Eenjun Hwang. Email:
(This article belongs to the Special Issue: Artificial Intelligence and IoT based intelligent systems using high performance computing for Medical applications.)
Computers, Materials & Continua 2021, 68(3), 2945-2959. https://doi.org/10.32604/cmc.2021.017435
Received 29 January 2021; Accepted 01 March 2021; Issue published 06 May 2021
Abstract
One popular strategy to reduce the enormous number of illnesses and deaths from a seasonal influenza pandemic is to obtain the influenza vaccine on time. Usually, vaccine production preparation must be done at least six months in advance, and accurate long-term influenza forecasting is essential for this. Although diverse machine learning models have been proposed for influenza forecasting, they focus on short-term forecasting, and their performance is too dependent on input variables. For a country’s long-term influenza forecasting, typical surveillance data are known to be more effective than diverse external data on the Internet. We propose a two-stage data selection scheme for worldwide surveillance data to construct a long-term forecasting model for influenza in the target country. In the first stage, using a simple forecasting model based on the country’s surveillance data, we measured the change in performance by adding surveillance data from other countries, shifted by up to 52 weeks. In the second stage, for each set of surveillance data sorted by accuracy, we incrementally added data as input if the data have a positive effect on the performance of the forecasting model in the first stage. Using the selected surveillance data, we trained a new long-term forecasting model for influenza and perform influenza forecasting for the target country. We conducted extensive experiments using six machine learning models for the three target countries to verify the effectiveness of the proposed method. We report some of the results.Keywords
Cite This Article
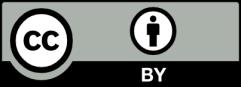
This work is licensed under a Creative Commons Attribution 4.0 International License , which permits unrestricted use, distribution, and reproduction in any medium, provided the original work is properly cited.