Open Access
ARTICLE
Data Matching of Solar Images Super-Resolution Based on Deep Learning
1 School of Information Engineering, Minzu University of China, Beijing, 100081, China
2 National Language Resource Monitoring and Research Center of Minority Languages, Minzu University of China, Beijing, 100081, China
3 CAS Key Laboratory of Solar Activity, National Astronomical Observatories, Beijing, 100101, China
4 Department of Physics, New Jersey Institute of Technology, Newark, New Jersey, 07102-1982, USA
* Corresponding Author: Song Wei. Email:
Computers, Materials & Continua 2021, 68(3), 4017-4029. https://doi.org/10.32604/cmc.2021.017086
Received 20 January 2021; Accepted 23 March 2021; Issue published 06 May 2021
Abstract
The images captured by different observation station have different resolutions. The Helioseismic and Magnetic Imager (HMI: a part of the NASA Solar Dynamics Observatory (SDO) has low-precision but wide coverage. And the Goode Solar Telescope (GST, formerly known as the New Solar Telescope) at Big Bear Solar Observatory (BBSO) solar images has high precision but small coverage. The super-resolution can make the captured images become clearer, so it is wildly used in solar image processing. The traditional super-resolution methods, such as interpolation, often use single image’s feature to improve the image’s quality. The methods based on deep learning-based super-resolution image reconstruction algorithms have better quality, but small-scale features often become ambiguous. To solve this problem, a transitional amplification network structure is proposed. The network can use the two types images relationship to make the images clear. By adding a transition image with almost no difference between the source image and the target image, the transitional amplification training procedure includes three parts: transition image acquisition, transition network training with source images and transition images, and amplification network training with transition images and target images. In addition, the traditional evaluation indicators based on structural similarity (SSIM) and peak signal-to-noise ratio (PSNR) calculate the difference in pixel values and perform poorly in cross-type image reconstruction. The method based on feature matching can effectively evaluate the similarity and clarity of features. The experimental results show that the quality index of the reconstructed image is consistent with the visual effect.Keywords
Cite This Article
Citations
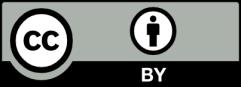
This work is licensed under a Creative Commons Attribution 4.0 International License , which permits unrestricted use, distribution, and reproduction in any medium, provided the original work is properly cited.