Open Access
ARTICLE
Bitcoin Candlestick Prediction with Deep Neural Networks Based on Real Time Data
1 Department of Information Technology, College of Computer, Qassim University, Buraydah, 51452, Saudi Arabia
2 Department of Computer Science, College of Computer, Qassim University, Buraydah, 51452, Saudi Arabia
* Corresponding Author: Amal A. Al-Shargabi. Email:
(This article belongs to the Special Issue: Artificial Intelligence and Big Data in Entrepreneurship)
Computers, Materials & Continua 2021, 68(3), 3215-3233. https://doi.org/10.32604/cmc.2021.016881
Received 14 January 2021; Accepted 26 February 2021; Issue published 06 May 2021
Abstract
Currently, Bitcoin is the world’s most popular cryptocurrency. The price of Bitcoin is extremely volatile, which can be described as high-benefit and high-risk. To minimize the risk involved, a means of more accurately predicting the Bitcoin price is required. Most of the existing studies of Bitcoin prediction are based on historical (i.e., benchmark) data, without considering the real-time (i.e., live) data. To mitigate the issue of price volatility and achieve more precise outcomes, this study suggests using historical and real-time data to predict the Bitcoin candlestick—or open, high, low, and close (OHLC)—prices. Seeking a better prediction model, the present study proposes time series-based deep learning models. In particular, two deep learning algorithms were applied, namely, long short-term memory (LSTM) and gated recurrent unit (GRU). Using real-time data, the Bitcoin candlesticks were predicted for three intervals: the next 4 h, the next 12 h, and the next 24 h. The results showed that the best-performing model was the LSTM-based model with the 4-h interval. In particular, this model achieved a stellar performance with a mean absolute percentage error (MAPE) of 0.63, a root mean square error (RMSE) of 0.0009, a mean square error (MSE) of 9e-07, a mean absolute error (MAE) of 0.0005, and an R-squared coefficient (R2) of 0.994. With these results, the proposed prediction model has demonstrated its efficiency over the models proposed in previous studies. The findings of this study have considerable implications in the business field, as the proposed model can assist investors and traders in precisely identifying Bitcoin sales and buying opportunities.Keywords
Cite This Article
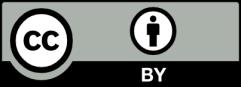