Open Access
ARTICLE
Video Analytics Framework for Human Action Recognition
1 Department of Computer Science, HITEC University Taxila, Taxila, 47080, Pakistan
2 College of Computer Science and Engineering, University of Ha’il, Ha’il, Saudi Arabia
3 Department of Electrical Engineering, College of Engineering, Jouf University, Sakaka, Saudi Arabia
4 College of Computer Engineering and Sciences, Prince Sattam Bin Abdulaziz University, Al-Khraj, Saudi Arabia
5 Department of Computer Science and Engineering, Soonchunhyang University, Asan, Korea
6 Department of Computer Science, COMSATS University Islamabad, Wah Campus, 47040, Pakistan
* Corresponding Author: Yunyoung Nam. Email:
(This article belongs to the Special Issue: Recent Advances in Deep Learning, Information Fusion, and Features Selection for Video Surveillance Application)
Computers, Materials & Continua 2021, 68(3), 3841-3859. https://doi.org/10.32604/cmc.2021.016864
Received 14 January 2021; Accepted 19 February 2021; Issue published 06 May 2021
Abstract
Human action recognition (HAR) is an essential but challenging task for observing human movements. This problem encompasses the observations of variations in human movement and activity identification by machine learning algorithms. This article addresses the challenges in activity recognition by implementing and experimenting an intelligent segmentation, features reduction and selection framework. A novel approach has been introduced for the fusion of segmented frames and multi-level features of interests are extracted. An entropy-skewness based features reduction technique has been implemented and the reduced features are converted into a codebook by serial based fusion. A custom made genetic algorithm is implemented on the constructed features codebook in order to select the strong and well-known features. The features are exploited by a multi-class SVM for action identification. Comprehensive experimental results are undertaken on four action datasets, namely, Weizmann, KTH, Muhavi, and WVU multi-view. We achieved the recognition rate of 96.80%, 100%, 100%, and 100% respectively. Analysis reveals that the proposed action recognition approach is efficient and well accurate as compare to existing approaches.Keywords
Cite This Article
M. Attique Khan, M. Alhaisoni, A. Armghan, F. Alenezi, U. Tariq et al., "Video analytics framework for human action recognition," Computers, Materials & Continua, vol. 68, no.3, pp. 3841–3859, 2021. https://doi.org/10.32604/cmc.2021.016864Citations
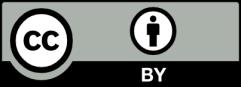