Open Access
ARTICLE
CARM: Context Based Association Rule Mining for Conventional Data
1 Faculty of Engineering & Information Technology, Foundation University, Islamabad, Pakistan
2 Department of Computer Science, Barani Institute of Information Technology, Rawalpindi, Pakistan
* Corresponding Author: Muhammad Shaheen. Email:
Computers, Materials & Continua 2021, 68(3), 3305-3322. https://doi.org/10.32604/cmc.2021.016766
Received 11 January 2021; Accepted 14 March 2021; Issue published 06 May 2021
Abstract
This paper is aimed to develop an algorithm for extracting association rules, called Context-Based Association Rule Mining algorithm (CARM), which can be regarded as an extension of the Context-Based Positive and Negative Association Rule Mining algorithm (CBPNARM). CBPNARM was developed to extract positive and negative association rules from Spatio-temporal (space-time) data only, while the proposed algorithm can be applied to both spatial and non-spatial data. The proposed algorithm is applied to the energy dataset to classify a country’s energy development by uncovering the enthralling interdependencies between the set of variables to get positive and negative associations. Many association rules related to sustainable energy development are extracted by the proposed algorithm that needs to be pruned by some pruning technique. The context, in this paper serves as a pruning measure to extract pertinent association rules from non-spatial data. Conditional Probability Increment Ratio (CPIR) is also added in the proposed algorithm that was not used in CBPNARM. The inclusion of the context variable and CPIR resulted in fewer rules and improved robustness and ease of use. Also, the extraction of a common negative frequent itemset in CARM is different from that of CBPNARM. The rules created by the proposed algorithm are more meaningful, significant, relevant and insightful. The accuracy of the proposed algorithm is compared with the Apriori, PNARM and CBPNARM algorithms. The results demonstrated enhanced accuracy, relevance and timeliness.Keywords
Cite This Article
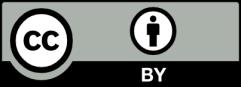
This work is licensed under a Creative Commons Attribution 4.0 International License , which permits unrestricted use, distribution, and reproduction in any medium, provided the original work is properly cited.