Open Access
ARTICLE
Suggestion Mining from Opinionated Text of Big Social Media Data
1 Department of Computer Science, College of Computer and Information Systems, Umm Al-Qura University, Saudi Arabia
2 Department of Computer Science, Faculty of Engineering and Computer Sciences, National University of Modern Languages, Islamabad, Pakistan
3 Department of Software Engineering, Faculty of Engineering and Computer Sciences, National University of Modern Languages, Islamabad, Pakistan
4 Department of Computer Sciences, Institute of Space Technology, Islamabad, Pakistan
5 Department of Computer Science, College of Computers and Information Technology, Taif University, Taif, 21944, Saudi Arabia
6 Department of Information Technology, College of Computers and Information Technology, Taif University, Taif, Saudi Arabia
* Corresponding Author: Youseef Alotaibi. Email:
Computers, Materials & Continua 2021, 68(3), 3323-3338. https://doi.org/10.32604/cmc.2021.016727
Received 09 January 2021; Accepted 15 March 2021; Issue published 06 May 2021
Abstract
Social media data are rapidly increasing and constitute a source of user opinions and tips on a wide range of products and services. The increasing availability of such big data on biased reviews and blogs creates challenges for customers and businesses in reviewing all content in their decision-making process. To overcome this challenge, extracting suggestions from opinionated text is a possible solution. In this study, the characteristics of suggestions are analyzed and a suggestion mining extraction process is presented for classifying suggestive sentences from online customers’ reviews. A classification using a word-embedding approach is used via the XGBoost classifier. The two datasets used in this experiment relate to online hotel reviews and Microsoft Windows App Studio discussion reviews. F1, precision, recall, and accuracy scores are calculated. The results demonstrated that the XGBoost classifier outperforms—with an accuracy of more than 80%. Moreover, the results revealed that suggestion keywords and phrases are the predominant features for suggestion extraction. Thus, this study contributes to knowledge and practice by comparing feature extraction classifiers and identifying XGBoost as a better suggestion mining process for identifying online reviews.Keywords
Cite This Article
Citations
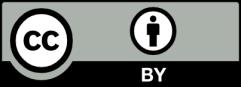
This work is licensed under a Creative Commons Attribution 4.0 International License , which permits unrestricted use, distribution, and reproduction in any medium, provided the original work is properly cited.