Open Access
ARTICLE
Unsupervised Domain Adaptation Based on Discriminative Subspace Learning for Cross-Project Defect Prediction
1 Nanjing University of Posts and Telecommunications, Nanjing, 210003, China
2 Jiangsu Engineering Research Center of HPC and Intelligent Processing, Nanjing, 210003, China
3 Wuhan University, Wuhan, 430072, China
4 Nanjing University of Information Science and Technology, Nanjing, 210044, China
5 Carnegie Mellon University, Pittsburgh, 15213, USA
* Corresponding Author: Yanfei Sun. Email:
Computers, Materials & Continua 2021, 68(3), 3373-3389. https://doi.org/10.32604/cmc.2021.016539
Received 04 January 2021; Accepted 26 February 2021; Issue published 06 May 2021
Abstract
Cross-project defect prediction (CPDP) aims to predict the defects on target project by using a prediction model built on source projects. The main problem in CPDP is the huge distribution gap between the source project and the target project, which prevents the prediction model from performing well. Most existing methods overlook the class discrimination of the learned features. Seeking an effective transferable model from the source project to the target project for CPDP is challenging. In this paper, we propose an unsupervised domain adaptation based on the discriminative subspace learning (DSL) approach for CPDP. DSL treats the data from two projects as being from two domains and maps the data into a common feature space. It employs cross-domain alignment with discriminative information from different projects to reduce the distribution difference of the data between different projects and incorporates the class discriminative information. Specifically, DSL first utilizes subspace learning based domain adaptation to reduce the distribution gap of data between different projects. Then, it makes full use of the class label information of the source project and transfers the discrimination ability of the source project to the target project in the common space. Comprehensive experiments on five projects verify that DSL can build an effective prediction model and improve the performance over the related competing methods by at least 7.10% and 11.08% in terms of G-measure and AUC.Keywords
Cite This Article
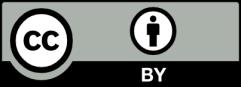
This work is licensed under a Creative Commons Attribution 4.0 International License , which permits unrestricted use, distribution, and reproduction in any medium, provided the original work is properly cited.