Open Access
ARTICLE
Reinforcement Learning-Based Optimization for Drone Mobility in 5G and Beyond Ultra-Dense Networks
1 School of Optical Engineering, Sejong University, Seoul, 05006, Korea
2 School of Intelligent Mechatronics Engineering, Sejong University, Seoul, 05006, Korea
* Corresponding Author: Ajung Kim. Email:
Computers, Materials & Continua 2021, 68(3), 3807-3823. https://doi.org/10.32604/cmc.2021.016087
Received 22 December 2020; Accepted 23 February 2021; Issue published 06 May 2021
Abstract
Drone applications in 5th generation (5G) networks mainly focus on services and use cases such as providing connectivity during crowded events, human-instigated disasters, unmanned aerial vehicle traffic management, internet of things in the sky, and situation awareness. 4G and 5G cellular networks face various challenges to ensure dynamic control and safe mobility of the drone when it is tasked with delivering these services. The drone can fly in three-dimensional space. The drone connectivity can suffer from increased handover cost due to several reasons, including variations in the received signal strength indicator, co-channel interference offered to the drone by neighboring cells, and abrupt drop in lobe edge signals due to antenna nulls. The baseline greedy handover algorithm only ensures the strongest connection between the drone and small cells so that the drone may experience several handovers. Intended for fast environment learning, machine learning techniques such as Q-learning help the drone fly with minimum handover cost along with robust connectivity. In this study, we propose a Q-learning-based approach evaluated in three different scenarios. The handover decision is optimized gradually using Q-learning to provide efficient mobility support with high data rate in time-sensitive applications, tactile internet, and haptics communication. Simulation results demonstrate that the proposed algorithm can effectively minimize the handover cost in a learning environment. This work presents a notable contribution to determine the optimal route of drones for researchers who are exploring UAV use cases in cellular networks where a large testing site comprised of several cells with multiple UAVs is under consideration.Keywords
Cite This Article
Citations
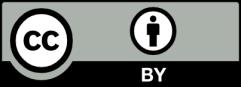