Open Access
ARTICLE
A Novel Deep Neural Network for Intracranial Haemorrhage Detection and Classification
1 Department of ECE, KPR Institute of Engineering and Technology, Coimbatore, 641407, India
2 Department of Electronics and Communication Engineering, University College of Engineering, BIT Campus, Anna University, Tiruchirappalli, 620024, India
3 Department of Medical Equipment Technology, College of Applied Medical Sciences, Majmaah University, Al Majmaah, 11952, Saudi Arabia
4 Department of Entrepreneurship and Logistics, Plekhanov Russian University of Economics, Moscow, 117997, Russia
5 Department of Logistics, State University of Management, Moscow, 109542, Russia
6 Department of Computer Applications, Alagappa University, Karaikudi, 630001, India
* Corresponding Author: K. Shankar. Email:
Computers, Materials & Continua 2021, 68(3), 2877-2893. https://doi.org/10.32604/cmc.2021.015480
Received 24 November 2020; Accepted 28 February 2021; Issue published 06 May 2021
Abstract
Data fusion is one of the challenging issues, the healthcare sector is facing in the recent years. Proper diagnosis from digital imagery and treatment are deemed to be the right solution. Intracerebral Haemorrhage (ICH), a condition characterized by injury of blood vessels in brain tissues, is one of the important reasons for stroke. Images generated by X-rays and Computed Tomography (CT) are widely used for estimating the size and location of hemorrhages. Radiologists use manual planimetry, a time-consuming process for segmenting CT scan images. Deep Learning (DL) is the most preferred method to increase the efficiency of diagnosing ICH. In this paper, the researcher presents a unique multi-modal data fusion-based feature extraction technique with Deep Learning (DL) model, abbreviated as FFE-DL for Intracranial Haemorrhage Detection and Classification, also known as FFEDL-ICH. The proposed FFEDL-ICH model has four stages namely, preprocessing, image segmentation, feature extraction, and classification. The input image is first preprocessed using the Gaussian Filtering (GF) technique to remove noise. Secondly, the Density-based Fuzzy C-Means (DFCM) algorithm is used to segment the images. Furthermore, the Fusion-based Feature Extraction model is implemented with handcrafted feature (Local Binary Patterns) and deep features (Residual Network-152) to extract useful features. Finally, Deep Neural Network (DNN) is implemented as a classification technique to differentiate multiple classes of ICH. The researchers, in the current study, used benchmark Intracranial Haemorrhage dataset and simulated the FFEDL-ICH model to assess its diagnostic performance. The findings of the study revealed that the proposed FFEDL-ICH model has the ability to outperform existing models as there is a significant improvement in its performance. For future researches, the researcher recommends the performance improvement of FFEDL-ICH model using learning rate scheduling techniques for DNN.Keywords
Cite This Article
Citations
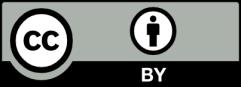