Open Access
ARTICLE
Modelling Intelligent Driving Behaviour Using Machine Learning
1 School of Computer Science, NCBA&E, Lahore, 54000, Pakistan
2 Riphah School of Computing & Innovation, Faculty of Computing, Riphah International University, Lahore Campus, Lahore, 54000, Pakistan
3 Department of Computer Science, Lahore Garrison University, Lahore, 54000, Pakistan
4 Department of Computer Science, College of Computer and Information Sciences, Jouf University, Skaka, Aljouf, 72341, Saudi Arabia
5 Department of Computer Science, Faculty of Computers and Artificial Intelligence, Cairo University, 12613, Egypt
* Corresponding Author: Muhammad Adnan Khan. Email:
Computers, Materials & Continua 2021, 68(3), 3061-3077. https://doi.org/10.32604/cmc.2021.015441
Received 21 November 2020; Accepted 04 March 2021; Issue published 06 May 2021
Abstract
In vehicular systems, driving is considered to be the most complex task, involving many aspects of external sensory skills as well as cognitive intelligence. External skills include the estimation of distance and speed, time perception, visual and auditory perception, attention, the capability to drive safely and action-reaction time. Cognitive intelligence works as an internal mechanism that manages and holds the overall driver’s intelligent system.These cognitive capacities constitute the frontiers for generating adaptive behaviour for dynamic environments. The parameters for understanding intelligent behaviour are knowledge, reasoning, decision making, habit and cognitive skill. Modelling intelligent behaviour reveals that many of these parameters operate simultaneously to enable drivers to react to current situations. Environmental changes prompt the parameter values to change, a process which continues unless and until all processes are completed. This paper model intelligent behaviour by using a ‘driver behaviour model’ to obtain accurate intelligent driving behaviour patterns. This model works on layering patterns in which hierarchy and coherence are maintained to transfer the data with accuracy from one module to another. These patterns constitute the outcome of different modules that collaborate to generate appropriate values. In this case, accurate patterns were acquired using ANN static and dynamic non-linear autoregressive approach was used and for further accuracy validation, time-series dynamic backpropagation artificial neural network, multilayer perceptron and random sub-space on real-world data were also applied.Keywords
Cite This Article
Citations
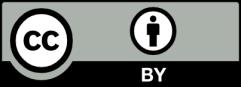
This work is licensed under a Creative Commons Attribution 4.0 International License , which permits unrestricted use, distribution, and reproduction in any medium, provided the original work is properly cited.