Open Access
ARTICLE
A New Hybrid Feature Selection Method Using T-test and Fitness Function
1 Department of Computer Science, Faculty of Science, University of Baghdad, Baghdad, Iraq
2 Faculty of Computer Science, Technology University, Baghdad, Iraq
3 Department of Computer Science, Al-Turath University College, Baghdad, Iraq
* Corresponding Author: Husam Ali Abdulmohsin. Email:
(This article belongs to the Special Issue: AI, IoT, Blockchain Assisted Intelligent Solutions to Medical and Healthcare Systems)
Computers, Materials & Continua 2021, 68(3), 3997-4016. https://doi.org/10.32604/cmc.2021.014840
Received 21 October 2020; Accepted 08 March 2021; Issue published 06 May 2021
Abstract
Feature selection (FS) (or feature dimensional reduction, or feature optimization) is an essential process in pattern recognition and machine learning because of its enhanced classification speed and accuracy and reduced system complexity. FS reduces the number of features extracted in the feature extraction phase by reducing highly correlated features, retaining features with high information gain, and removing features with no weights in classification. In this work, an FS filter-type statistical method is designed and implemented, utilizing a t-test to decrease the convergence between feature subsets by calculating the quality of performance value (QoPV). The approach utilizes the well-designed fitness function to calculate the strength of recognition value (SoRV). The two values are used to rank all features according to the final weight (FW) calculated for each feature subset using a function that prioritizes feature subsets with high SoRV values. An FW is assigned to each feature subset, and those with FWs less than a predefined threshold are removed from the feature subset domain. Experiments are implemented on three datasets: Ryerson Audio-Visual Database of Emotional Speech and Song, Berlin, and Surrey Audio-Visual Expressed Emotion. The performance of the F-test and F-score FS methods are compared to those of the proposed method. Tests are also conducted on a system before and after deploying the FS methods. Results demonstrate the comparative efficiency of the proposed method. The complexity of the system is calculated based on the time overhead required before and after FS. Results show that the proposed method can reduce system complexity.
Keywords
Cite This Article
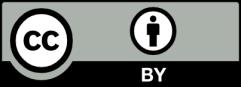