Open Access
ARTICLE
Segmentation of Brain Tumor Magnetic Resonance Images Using a Teaching-Learning Optimization Algorithm
1 Department of Computer Science and Engineering, Sona College of Technology, Salem, 636005, Tamilnadu, India
2 Department of Electronics and Communication Engineering, K. Ramakrishnan College of Technology, Trichy, 621112, India
3 Department of Electronics and Communication Engineering, University College of Engineering, Anna University, Tiruchirappalli, 620024, India
4 Department of Mechanical Engineering, Rohini College of Engineering and Technology, Palkulam, 629401, India
5 Department of Electrical and Electronics Engineering, National Engineering College, Kovilpatti, 628503, India
6 Department of Medical Equipment Technology, College of Applied Medical Sciences, Majmaah University, AlMajmaah, 11952, Kingdom of Saudi Arabia
7 Department of Electrical and Electronics Engineering, SRM Institute of Science and Technology, Chennai, 603203, India
* Corresponding Author: J. Jayanthi. Email:
Computers, Materials & Continua 2021, 68(3), 4191-4203. https://doi.org/10.32604/cmc.2021.012252
Received 30 August 2020; Accepted 13 March 2021; Issue published 06 May 2021
Abstract
Image recognition is considered to be the pre-eminent paradigm for the automatic detection of tumor diseases in this era. Among various cancers identified so far, glioma, a type of brain tumor, is one of the deadliest cancers, and it remains challenging to the medicinal world. The only consoling factor is that the survival rate of the patient is increased by remarkable percentage with the early diagnosis of the disease. Early diagnosis is attempted to be accomplished with the changes observed in the images of suspected parts of the brain captured in specific interval of time. From the captured image, the affected part of the brain is analyzed using magnetic resonance imaging (MRI) technique. Existence of different modalities in the captured MRI image demands the best automated model for the easy identification of malignant cells. Number of image processing techniques are available for processing the images to identify the affected area. This study concentrates and proposes to improve early diagnosis of glioma using a preprocessing boosted teaching and learning optimization (P-BTLBO) algorithm that automatically segments a brain tumor in an given MRI image. Preprocessing involves contrast enhancement and skull stripping procedures through contrast limited adaptive histogram equalization technique. The traditional TLBO algorithm that works with the perspective of teacher and the student is here improved by using a boosting mechanism. The results obtained using this P-BTLBO algorithm is compared on different benchmark images for the validation of its standard. The experimental findings show that P-BTLBO algorithm approach outperforms other existing algorithms of its kind.Keywords
Cite This Article
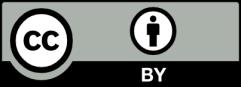