Open Access
ARTICLE
Deep-Learning-Empowered 3D Reconstruction for Dehazed Images in IoT-Enhanced Smart Cities
1 Department of Computer Science and Technology, Xi’an University of Science and Technology, Xi’an, 710054, China
2 Tamoritsusho Co., Ltd., Tokyo, 110-0005, Japan
3 Global Information and Telecommunication Institute, Waseda University, Tokyo, 169-8050, Japan
4 School of Fundamental Science and Engineering, Waseda University, Tokyo, 169-8050, Japan
* Corresponding Author: Xin Qi. Email:
(This article belongs to the Special Issue: Deep Learning and Parallel Computing for Intelligent and Efficient IoT)
Computers, Materials & Continua 2021, 68(2), 2807-2824. https://doi.org/10.32604/cmc.2021.017410
Received 29 January 2021; Accepted 01 March 2021; Issue published 13 April 2021
Abstract
With increasingly more smart cameras deployed in infrastructure and commercial buildings, 3D reconstruction can quickly obtain cities’ information and improve the efficiency of government services. Images collected in outdoor hazy environments are prone to color distortion and low contrast; thus, the desired visual effect cannot be achieved and the difficulty of target detection is increased. Artificial intelligence (AI) solutions provide great help for dehazy images, which can automatically identify patterns or monitor the environment. Therefore, we propose a 3D reconstruction method of dehazed images for smart cities based on deep learning. First, we propose a fine transmission image deep convolutional regression network (FT-DCRN) dehazing algorithm that uses fine transmission image and atmospheric light value to compute dehazed image. The DCRN is used to obtain the coarse transmission image, which can not only expand the receptive field of the network but also retain the features to maintain the nonlinearity of the overall network. The fine transmission image is obtained by refining the coarse transmission image using a guided filter. The atmospheric light value is estimated according to the position and brightness of the pixels in the original hazy image. Second, we use the dehazed images generated by the FT-DCRN dehazing algorithm for 3D reconstruction. An advanced relaxed iterative fine matching based on the structure from motion (ARI-SFM) algorithm is proposed. The ARI-SFM algorithm, which obtains the fine matching corner pairs and reduces the number of iterations, establishes an accurate one-to-one matching corner relationship. The experimental results show that our FT-DCRN dehazing algorithm improves the accuracy compared to other representative algorithms. In addition, the ARI-SFM algorithm guarantees the precision and improves the efficiency.Keywords
Cite This Article
Citations
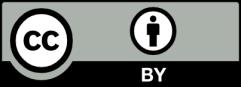
This work is licensed under a Creative Commons Attribution 4.0 International License , which permits unrestricted use, distribution, and reproduction in any medium, provided the original work is properly cited.